#8: Music Recommender Systems, Fairness and Evaluation with Christine Bauer
Note: This transcript has been generated automatically using OpenAI's whisper and may contain inaccuracies or errors. We recommend listening to the audio for a better understanding of the content. Please feel free to reach out if you spot any corrections that need to be made. Thank you for your understanding.
The set of artists were very clear about was, they said there is gender imbalance in the music business in general for decades.
And I found it really interesting.
They were so clear about maybe we can use recommender systems as a way to change that.
So finally as a solution.
And for me as a researcher, there was a very great moment to see like, okay, it's not only using it and having to live as a recommender, but seeing it as a solution to a problem that existed already beforehand.
Even a person is not the same the next day.
So it depends on the situation, on the intent, on the context, what is really relevant in the very moment.
If in this situation, the system has to decide, should it be track A or B that's played, and for the consumer, it wouldn't make a difference, but it could help to like increase fairness or to give someone a chance or whatever the other reasoning behind that is, then it's an easy possibility to like flip it around.
We need to take different perspectives to get the full picture from the evolution and not only zoom in one aspect and ignore what else is happening.
And then we don't know a lot about the recommender.
Hello and welcome to this new episode of RECSPERTS recommender systems experts.
In this episode, we are talking about music recommender systems, fairness in music recommender systems, as well as the different complexities and challenges around the evaluation of recommender systems.
And today's guest is maybe a name that you have heard about in one of the previous episodes, because my guest today is Christine Bauer.
Christine Bauer is assistant professor at the University of Utrecht in the Netherlands, and she is researching music recommender systems quite a lot and has some dedicated interest for the fairness of recommender systems.
She has studied in Vienna at the Technical University over there as well as the University of Vienna and spent some research at the Carnegie Mellon University, as well as some time in Cologne and Linz.
With papers being submitted to different journals and UMAP, RecSys or CHI or dub dub dub, she has also shown many different contributions in the field of recommender systems.
And especially in the RecSys community, she is known for co-organizing the Doctorial Symposium as well as the perspectives workshops that will again take place this year.
Welcome Christine Bauer.
Hello, welcome.
Thanks for the nice introduction.
Yeah, it's nice to have you on this show and to finally talk to you because I guess there was not only a single person who recommended you.
I mean, we knew before from the RecSys and so it's great finally talking to you in this episode.
Lots of pressure on me, as many people recommend me, but in like recommender systems we have to recommend.
Definitely.
Do you like to give us just some overview about yourself and your work in recommender systems?
That's a challenging start.
Yeah, that's a challenging start.
Recommender systems, I actually started only in 2017 to really dedicate my research to recommender systems.
I worked before on different topics and the last one where I was really delving into was context aware computing, context aware systems, where it didn't focus on recommender systems at all, but it was a very, very straightforward way to flip over to recommender systems.
And as I'm so much interested in music, it's even more pleasure to apply these things to the music domain.
And that's where I think I'm most known for doing research in the music recommenders field.
But actually my field is much broader, so I'm interested in fairness.
How can we create fair recommenders?
What is the impact of recommenders?
And that's the reason why we need fair recommenders.
And the big challenge that we generally have is how do we evaluate?
Well, we evaluate the systems, but do we do it in a way that is okay?
And yeah, sneak peek, no.
Surprise, surprise.
What a surprise.
So I see that as an overarching theme that I follow and really want to push forward.
And that's not only relevant in the music recommenders field.
Okay, yeah, that definitely makes sense.
So not only there, not only tied to entertainment, but also to very different domains like e-commerce or social media, where I guess we deal a lot with fairness and other aspects of recommendations apart from pure accuracy of retrieval.
Yeah, that's actually a point that you address that music is associated with entertainment because as a consumer, we want to be entertained.
But it's a big ecosystem where lots of stakeholders are in place.
And especially for the artists, it's not, oh, it's entertainment.
They want or need to make a living of it.
So there are different interests in there.
So it's not only entertainment, but it's the first thing we associate with such a domain.
Yeah, it's mainly from a user centric point, it would be entertainment.
But of course, artists and also, that's for example, record selling companies want to live from something and pay their employees from something and need to earn money with it and of course, also the artists.
Yeah, so why actually music recommender systems or let's just take a step back.
Why recommender systems at all?
What was it that brought you into recommender systems?
I think there is not a one straightforward answer to that.
So the one thing was, and I mentioned that already, that I was researching on context aware systems and particularly addressed it in the advertising field.
And this has sometimes this bad connotation or advertising is for making people buy things that they actually don't want and don't need, which is not always the case.
But sometimes that's the case.
But that has a bad aftertaste when you do research in the field.
And I was watching out for other domains.
And I was like, yeah, I like music a lot.
I start playing instruments when at the age of four and I worked at the Collecting Society in Austria music domain for performing rights and I'm still playing and I know lots of musicians, some labels and so on.
So I am really strongly attached to this domain and I really like that I could combine the things altogether.
And I was doing research in lots of different fields where music was somehow in play, for instance, how can a chugging app adapt with the music it's playing so that you can do better performance in your training?
So music was already in there or how do artists learn the management skills that they need?
So the music or music domain was always in there, although I really addressed lots of different topics and somehow in the end it all came together and it's music recommenders.
So that means you really know what you talk about when it comes to artists and the artists' perspectives on recommender systems because you are basically doing music or playing music and in that sense also an artist yourself when it comes to music, right?
Or a statement, but hopefully I know what I'm talking about, but I definitely have background that other people don't have and that helps in doing research in this field.
Yeah, I guess it's always kind of nice if you also have some kind of personal attachment to the work that you perform and if you say that you like to create music and like to play certain instruments and something like that, then this is kind of a personal attachment that you're having and if you find that also resembled on parts of your work, then I guess that makes work also sometimes much more joyful.
It's more joyful, it's also motivating just because of that, but also when you have the background you know lots of stakeholders, how they're involved, how they're affected, and it's easier to see the need or the impact and that's again a driver to move forward and make the next step.
When talking about music recommender systems and especially, I mean, there are many platforms in the domain that we have mentioned in previous episodes.
These platforms have interests and the users have interests and the artists have interest just to name a couple of the involved stakeholders.
What is the problem in balancing those interests and can you just give us a broad overview about potential approaches of balancing those interests?
Okay, you're specifically asking for the music domain.
Yes, for the music domain.
Okay, what's the challenge?
The lots of challenges involved, well, there are several stakeholders, you named them already, but there is like the different, the power relationships are very imbalanced ever since in the music business long before recommender systems and of course, these power imbalances still alive.
And when we think about like these platforms, they have recommender systems in there who will decide what's recommended and how and what's implemented and some stakeholders have more say in that like the platform owners themselves, of course, than the various artists.
For artists, for instance, it's not like one big stakeholder, it's like lots of different individuals or small groups and they're also there different power like we have the super, super stars and then we have some people just release one item, which is good or not good, we don't know.
And then there's a wide scale of in between.
We know that there's this long tail distribution of popularity and that of course reflects like the power that people have in the system, but also influence the recommender systems, how we, how we form them.
And then again, we have some stakeholders as aggregators or as middle entities, for instance, some record companies, they usually don't represent only one artist.
There are small labels that do it like that, then it's typically the artist themselves representing them.
But usually a record company represents lots of artists with lots of different, again, different power relationships, different prospects of what could come out in terms of popularity, in terms of money, in terms of whatever.
So that's already the starting point before even a recommender is in place.
Yeah.
Yeah.
So do you think that, for example, record companies, I mean, you said that they are one of the powerful stakeholders in that overall system.
Do they think that they have a saying when it comes to recommender systems or kind of, I wouldn't say dictating, but influencing the decision of how personalization is being done by platforms?
That's a good question.
Well, you have to ask them themselves if they think they can influence that.
It's like, whatever I say, it's just a, could only be a rumor.
But it would be, I can phrase it like that.
It would be a surprise if there's no influence at all.
I would be very surprised.
Then this would mean that the platform almost have even more power than I thought.
Yeah.
Oh, that's right.
And they already have a lot.
Of course, it's their platform.
So yeah, a tricky question, but you have to direct it to someone else, I think.
Yeah, we'll take a note for that.
So maybe to be addressed in one of the upcoming episodes.
In music recommender systems, from a user perspective, we are mainly thinking about to get some recommendations, for example, to be resembled in a playlist and to have as many, let's say, relevant songs there as possible.
And I mean, relevant could mean different things.
It could be something that I haven't listened to for quite a long time, or also something that I haven't listened to yet.
And yeah, that I find novel and entertaining and also enriching in a sense that it adds some new direction for how my music taste might evolve in the future.
So we have even if we would ignore or disregard all the other stakeholders, I mean, there are already so many goals that only cater the user.
How are we going about these different goals and bring them into balance before even going into respecting the other stakeholders?
Yes, that's already the one big challenge, just considering one stakeholder.
Of course, I don't know what exactly is done at the platforms, but I assume that they make clear distinctions between user types, according to user types now, how they use a platform and how they want to use it, which might not be overlapping and concerning what you mentioned before that some people want to discover new things and like discover weekly, for instance, by one platform is a good place to go to where it's new and at the same time, hopefully relevant or matches the taste.
Some people just they're stuck in their they have formed their tastes.
They have their the songs that they like a huge collection and they're not really like sometimes something new comes in.
It's fine, but they're more attached to the old songs and kind of recycle them in new playlists.
So familiarity is a bigger goal there or something that's more important.
And what I think plays also plays a role is the right mixture because there might be users that always want to get something new.
But if I just think of myself, yes, I want to learn about new stuff, but it has to be sneaked in into something that is already very familiar.
And it also has to be in like the transition from one genre to another.
So for some people, they're like stuck to one genre or two genres.
And some people like have a very wide spectrum in their taste.
And again, for some people, it needs to be like a smoother transition between genres.
And for others, it's OK to have some soft song and death metal and some classical music and then reggae.
I'm sometimes OK with that and sometimes I'm not.
And that's to another point because even a person is not the same the next day.
So it depends on the situation, on the intent, on the context, what is really relevant in the very moment.
You just mentioned that about the running case.
And I just recall that there are also those playlists that target for a certain steps per minute or beats per minute level of your exercising session, especially, for example, when you are running, then there are these 160 BPM power a lot playlists and those that are maybe a bit slower.
So 120 or 130 BPM.
So of course, this is relatively explicit because when I know what kind of exercising session I want to perform as a runner, then I would explicitly select the corresponding playlist.
But what mechanisms or methods exist to capture this more implicitly?
I mean, you said I'm not the same person tomorrow as I am today.
So how am I going to catch personality change to name it or the change in context that then, of course, is going to determine my taste for the other day or the difference in taste?
Yeah, there are different approaches.
One way would be like explicitly asking for something and they're sometimes like asking for the mood.
Difficulties, people sometimes can't say what their mood is.
It's like other people can see it and feel it and yourself don't manage to express it correctly.
So that would be one way or what are you up to today via here at the platform.
So that this would be ways to explicitly ask with some predefined categories.
Another way would be as you said, implicitly trying to capture it from the way how people navigate a platform if the search for something and there are some papers already out into like this capturing the intent by someone at the moment at the platform.
For instance, if you search for a specific artist, then it's very likely that really want to know something about the artist, but someone could also like click through the old playlists until they reach the artist that they were searching for and then move from there.
So there are different ways and it's a challenge like how to find the different ways, how to deal with it.
And there's another challenge like with intent, it's already a challenge to find out what is the intent of a person when arriving at the platform, but it's typically not that the person has an intent and it stays like that.
And only the next time they arrive at the platform, it's a different one because it changes because you encounter something and then you deviate from it's like you're browsing on the web and suddenly you end up somewhere else and you don't even know why you ended up there, but somehow something changed in your journey and you were interested in something else.
And that's also in navigating lots of different platforms.
Yeah, I think there will be lots of research also in this field coming up, but we are not there yet to know what's going on.
People are complex.
So for example, one of my colleagues might recommend me or certain artists and then I directly after the daily go to my app and search for that artist.
So this might be something that is just given the features that we assume these platforms have access to totally unpredictable because I think they don't have access to what my colleague has kind of recommended me within the daily.
But this is basically at that very moment driving my intent to search for that artist and maybe listen to that artist.
Right.
Yes.
And especially here, like there's something happening in the background.
Nobody like nobody knows, hopefully like web cameras.
We don't know, please don't do it if like someone's listening, don't do it.
Yeah, but that's the thing.
Like there's something happening in the background.
There's like no information about that.
There's just like black box or like just time spent assume that nothing's happened, but something happened.
But I think as human creatures, like we sometimes it's not so mysterious what we're doing.
Like if if someone sees something on TV or someone else mentions this particular song or you just like remember the song for whatever reason and then you search for it.
Why do you enter a platform and directly search for a specific term something happened before that directed you exactly to that?
Yeah.
So it's not so much of a surprise, but you don't know what exactly happened.
So at least given the data of that very specific platform and that very example.
So no one knows why, but of course the behavior looks like there is some very specific intent the user had at that very moment.
Yeah.
Or for instance, like if someone like listens to something like three seconds and goes to the next one, it goes to the next one, it's browsing, but maybe it was a more specific intent and nothing matched it beforehand, which is different to like listening to a longer sequences and then moving to the next song.
There might be something different behind that.
So already from that we could infer something.
However, it's called in the end, but as long as the recommendations will fit that then it's fine.
Okay.
So I mean, the user is already quite complicated to grasp and there might be different truths for a user on different days.
I mean, we talked about that discovery aspect as well, but taking a broader look at it again, the word fairness, I would say has been used quite a lot in the past years because it has seen good development.
It has seen a rise in the considerations of ML practitioners, ML researchers, whether it might be in recommender systems or other application areas like vision.
What exactly do we mean when we talk about fairness?
So what does it mean?
I would assume there are some people who mean that something is equitable and there might be other people who mean that something's equal and even between equal and equitable, there's a great gap or just a big difference.
So I think as researchers in our community, like everybody talks about something slightly different and we're not there yet that we have this one concept that we adhere to.
And I think it will never be that there's one concept that's like worldwide generally over all the different domains.
There are definitely legal aspects that we have to consider and especially with discrimination law, we know what's not okay, which it's like flipping the coin and we know what's hopefully okay.
But there's also some subjectivity in it, what I consider fair might be that you don't consider that fair, but then we have to go into a discourse and find out, okay, how do we deal with that?
But it's not only about individuals, we have also the societal level and that makes it more complex.
And again, we are the different stakeholders at the different approaches, how we understand life or the meaning of life.
So it's all very complex and I already sneak in my topic with artists in fairness.
Yeah, please.
Let's make it more concrete.
So I was discussing with my colleague, Anders Ferrau, and he's also into music a lot and knows artists and was like, yeah, we think it's not fair for artists what's happening.
But then what's the question?
Yeah, but what's fair for artists?
And then, yeah, well, it's not on us to decide what's fair for artists.
We have to reach out to artists to ask them what's fair for you?
What do you want to have?
What do you need?
What's affecting you?
And that was the starting point of our journey of doing research together.
And we indeed reached out to artists with interviews and asked them how they perceive what's going on on platforms, on the music platforms and how they are affected, but also asked more concretely how they wanted to have the ideal recommender system to work for them.
Like for them as an artist, the role artist, but also in general for the music community.
And yeah, it was very interesting with lots of different viewpoints where again, we could see it's there's not one alignment, but there's some aspects that a lot of people mentioned, like for instance, gender balance was mentioned really a lot in the interviews.
Yeah, and that's a way how we try to come closer to understand what's fair.
And that's also how one paper starts with the article, what is fair to better understand what is the artist's perspective.
And we're continue working on that now also with my PhD, Kallen Dinesen, we explore more the artist's perspective on what they consider fair as fair and trying to find ways to put that into practice with the recommenders.
When talking to these artists, I would assume from different genres, from different popularity, and of course, different gender and so on and so forth.
Have they an understanding, I guess it's very different, of what is basically going on there when platforms offer their music in a personalized manner to users?
Or how was that very first step when asking about their perception and their demands with regards to fairness also talking about personalization and what's going on there?
So how was that exchange?
That's a very good point.
So like, as both Anders and me, we know quite some musicians and like, also of different popularity levels, it was pretty clear beforehand that there's different levels of understanding what's going on.
And so also for the interviews, we wanted to give them all the same basic level of a recommender is so we...
Here is your collaborative filtering 101 course.
More or less, yes, more or less, like in very simple terms, also with examples from the music domain.
There was a lot of, ah, okay, I didn't know.
So already there, the feedback was okay, yeah, we could get an understanding that it's not so clear, which is actually not a surprise, but still.
They are concentrating on good music and we are concentrating on research or ML practitioners.
So it's just that we have different intents and different expertise.
So I mean, we are not blaming musicians to have not such a data or system literacy.
So of course, no, no, I didn't mean it like that.
It's just, yeah, it's not a surprise that it's not, they don't have the full understanding as a researcher has like researcher focusing on recommender systems, but at the same time, learning that they're really affected by a lot of things, but just didn't know what's exactly going on.
So transparency is like the lack of transparency is big issue.
And if you don't know, you just know there's some algorithms somewhere or more, or you don't really know, and then your song is recommended or not, and you have no agency, but even if if someone would say, yeah, you can do something, but you don't know how, what's working behind that, you have no agency can do anything.
That's a big point.
And there's also, yeah, it sounds weird to say educating people because educating also sometimes has a bad aftertaste, but it's not necessary like to let people know what's going on.
So I'm not interested, like not all of them, but many were very interested to finding out what's going on, both from the artist perspective, but also the use platforms also as a consumer.
And that was also interesting to see in the interviews, we asked them to answer in the role as an artist, but we had to remind them sometimes because of course, you also use it as a private person and you have interest and it's mixing up and it's totally makes sense.
So, yeah.
So what was the outcome of these interviews?
I guess it's not a unanimous voice that directly tells you what fairness is, how to measure it and just go forward.
But if you would go for a slight conclusion, even though opinions might be diverse, what would that be?
Yeah, so it's still ongoing, like we have published on that, but we are continuing on that.
And in the first round of interviews was with Spanish speaking artists from different countries.
Their voice said, actually they don't want that the users taste and preferences are influenced or like purposefully influenced.
And there is no memory mark, but it somehow it's influenced anyways.
But where the set of artists were very clear about was they said there is gender imbalance in the music business in general for decades.
And I found it really interesting.
They were so clear about maybe we can use recommender systems as a way to change that.
So finally as a solution.
And for me as a researcher, that was a very great moment to see like, okay, it's not only using it and having to live as a recommender, but seeing it as a solution to a problem that existed already beforehand.
And yeah, so that was very interesting.
And it was also for me, interesting, like that the gender aspect like popped up in every interview.
We also in the interviews we made it really clear that we don't like ask everyone directly first, what do you think about gender imbalance because then it's expected what people say.
But rather like in general talk about how they want to have recommenders and then the gender topic popped up for some, it was the part of the discussion.
And that was the starting point when we then looked into algorithms and how we could use simple re-ranking to use it in a simulation to see how would it evolve over time?
Can gender balance be reached at all?
Does it do anything?
In the simulation, we could break the loop and increase the proportion of women that are recommended or songs by women that are recommended.
But again, it's computational simulation.
It's not a real world setting.
And that's what's needed in the end.
Yeah, yeah.
This is already interesting because it brings us maybe more to the metrics or to concrete measurements as at least try to quantify the impact of, let's say, fairness directed interventions in a recommender system if we assume that before intervening my recommender system would be purely focused on retrieval accuracy, for example.
I mean, by this you already imply what might be a notion of fairness.
So it might be that we are given an attribute, the attribute might be the gender and that fair is when, for example, the consumption spreads more evenly across genders.
There I sometimes, so I would sometimes question this a bit.
So because this is one of these notions where it says, okay, it's fair when it's equal.
But when looking at the, let's say, most popular artists, and we see that there are, of course, women there are men on the stage that are performing very greatly.
I mean, just last week and Lady Gaga has been performing in Dusseldorf and I guess we can acknowledge that she's one of, I mean, you don't need to like her music or something like that, but we can acknowledge that she is one of the most skilled entertainers and musicians of our time.
And then there we already see the point that making something equal is maybe also not right because we want to somehow also acknowledge the, let's say, the skill that musicians have or something like that.
And there, of course, we would assume that this is foundationally also equally distributed.
What is the goal at some point if we want to make something more fair?
So is it really equity?
So are we reaching for, I mean, we have, let's say, 10,000 artists and just if the consumption of music spreads evenly across these 10,000 artists, is then something regarded as fair?
So when would you say that something is fair?
Is it when your Gini coefficient is lower than 0.3?
Or how do we measure it in these kinds?
Or is it only fair if it's equal?
I don't think that fairness means equal, like equal and like lots of different aspects that we have to take into account and it's the package.
So if you only look into one metric, you don't know the big picture and there are sometimes trade offs and you have to look on everything.
So as a term that drives me, it's equal opportunities, which doesn't mean it has to be equally distributed in the end.
What I consider important is to look on what's happening now, trying to judge is that great or not?
And if it's not great, how should it be in which direction should it go?
So not how should it be, it should be 49 to 61 or 50-50 or 80-20, but in which direction should it go?
And then if it's acknowledged, not only your very own idea, trying to reach that.
And I think we will never have a system, whatever system in whatever domain that is fair, perfect.
We will never reach that, but it shouldn't mean that we stop doing anything in this direction, but it's a constant improvement, like working on it and pushing.
And yeah, that's, we're on the road and trying to change things.
And what you said before with having something like that's also why I use balanced and not having it equal because balance is more embracing.
But again, it depends on who you talk to, what it's all about, for instance, and it's just again, the gender topic.
So we could in our work only look on men and women because we only had data for that.
And there is a wide spectrum of genders and the non-binary spectrum is just not represented in the data.
And that was also a point for me where I said, okay, then it's not good if you can't use it, but not doing anything at all was also not, it didn't feel okay, but leaving out something is also not okay.
But then like I tell it myself, it's one step forward, acknowledging that it's not perfect at all and is also found it important to acknowledge that in the papers when you publish that and then trying to find ways to make it happen and to embrace a wider spectrum.
And that's just the attribute of gender and lots of different other aspects where we don't have even any data at all.
And we have to be creative in finding ways how we can create these equal opportunities.
So I'm really convinced on that, that we have to do that.
Or at least we don't have any public data on this.
So I guess the data is out there, but it's maybe not accessible.
Yeah, it's yeah, publicly accessible is one thing.
And yeah, for some aspects, there's a reason why it's not publicly accessible or it is not in one place altogether.
Because of the consequences.
Yeah.
Going back to your very study where you showed within that simulation that you could achieve a better balance.
How have you been measuring or quantifying that effect?
Okay, yeah.
So it's already with the balance, I said, it's a more generic term.
So what was particularly an issue for us, and that's actually a good sign that you have to look into the details.
It was not only the proportion of how many women or men were recommended, but also in what position.
So usually with recommendations, and particularly in music, it's not one song is recommended, and you listen to it and it's great.
And then you do something different.
It's typically you listen to several tracks.
And also how recommend those work, we do ranking.
So the high, like most accurate one is ranked first, and then the other ones come in.
For me to see in the data that it was like a woman always came on the roughly sevens position only, where the on average, and on average, the first man was number one.
That was an aspect.
It happened that we looked into that.
It's not 10 years of research to say that told us you have to look on the ranking.
It was something we explored and we were interested in.
And then we found, okay, that's something we have to look into because in the percentage, it was roughly the same as in the data set.
In general, roughly 25% are women and gender minorities among the artists.
And that was also represented in the recommendation.
So one could say, well, it's representing the input.
One could say that.
But if we consider that it has been like that for decades in the real world without recommenders.
And if you want to change it, we have to do something.
If you want to keep that, then it's fine.
But then again, we looked into detail.
Okay, what about the position in the ranking and also coverage, like how many tracks by women are actually recommended.
And that's specific for the music domain.
So there are quite some highly popular women.
You just mentioned before Lady Gaga, one of them.
And if you would use a popularity based approach, so recommending the most popular items to everyone, you have a high ratio of women in there because in the distribution, it's there like in the super superstars, there are quite some women.
And then I exaggerate now, long time, nothing.
And then in the low end of the popularity curve, there are lots of women again.
And you have to take this into consideration.
That's the input.
That's also the input.
And you have to deal with that.
Okay, okay, I see.
And so I'm definitely really surprised about that large gap in the rank.
And it's good that you're making that point.
It's a difference of saying, okay, but you're included in the top 10 or 20 recommendations, but you are not at a very high position on average.
These are just two different things.
And then you can't buy anything from just appearing within the list.
If you appear at the very bottom, I mean, seventh is not bottom, but compared to where men appear, then it's a big difference.
Yeah.
Then you somehow intervene.
So how did your intervention look like in that simulation?
So how do you create a system or change a system or a recommender such that the representation was becoming more balanced?
We took a very simple approach.
We used the ALS approach, what was computed there, took the output and then did re-ranking.
So we put men down in the ranking.
Like we tried out different approaches, like one position or five positions or seven positions and so on.
And then assumed in the simulation that users would consume what they're recommended in the top numbers and then retrained the model and then again, used the ALS approach and then applied the re-ranking again.
And yeah, that's a very simple approach.
Like re-ranking is a very simple approach.
And also assuming the, in the simulation that the top items are consumed is also rather simple, but we could already see the effect there.
And what I've also found interesting is that we could see that the number of items that had to be re-ranked, it decreased over the iterations.
So with the re-ranking, the original, I call it the original ALS approach already had the information that more women were listened to or like higher in the ranking.
To just get this right, you assume that things that have been appearing more up in the recommendation lists have been clicked or would have been clicked more.
So basically just set as a foundation the position bias there, right?
Which is, but it's based on research that indeed that's how we users, we as users act like the top, otherwise the position in the ranking wouldn't matter, but it matters because it's more likely that people consume what's shown first in the list.
Yeah.
Yeah.
I like the look at recommender systems in that multifaceted way that you do not only think of a recommender system, that it's a system that is kind of enforcing existing imbalances or existing unfairness, but that on the other side, it might also be as a tool that you could use in order to create more balance or create more fairness.
So it's the same thing, but just used in a different way to achieve certain things.
Yeah.
It came from the artist that he said, oh, we can use this to address a problem or a challenge that exists for a long time.
And that was very inspiring.
And how I try to continue on this path.
Perfect.
Cool.
If we go back to the users where we started from, how acceptable would they be with regards to recommender systems that not only take into account relevance of users, but that also takes into account fairness of, or fairness of artists or artist fairness, if you want to call it like that.
Sometimes this might also be like a trade off between these two things, not always.
So I'm not saying that in order to create artist fairness, you definitely need to decrease relevance of recommendations, but how is research dealing with that trade off or is there even a trade off or what is your point on this?
If you have these multiple objectives at play, there are lots of papers that I call it assume that there is definitely a trade off and I'm not convinced that there has to be a trade off.
Why?
Why are you not convinced of there being a trade off or what speaks against that there is a trade off?
Yeah, I'm not convinced that there's a trade off or that there has to be a trade off because there are lots of different things that come into play of what a person likes or accepts.
And for instance, in the music domain, there are certain tracks that you really, really like and without the track, you wouldn't be it.
But for certain situations, like if you want to do like do a meditation, have music in the background, does it really matter whether it's track A or track B?
A good point.
It could matter, especially if one of them is really bad, but in certain situations is somehow interchangeable from the perception of the consumer because it doesn't really matter in the very moment.
But if in this situation the system has to decide should it be track A or B that's played and for the consumer, it wouldn't make a difference, but it could help to like increase fairness or to give someone a chance or whatever the other reasoning behind that is, then it's an easy possibility to like flip it around.
Yeah, yeah, yeah.
And it depends on the situation and there are recommenders ways like you searching for the one and only item to purchase.
And then it needs to be the one thing and the most accurate one, that's the best one.
But for other fields and other situations, it's somehow interchangeable.
Actually have to think about my last concert.
Two weeks ago, I've been on a Rhett or Chili Peppers concert, which was great.
And there have been numerous bands playing before like you always have at some concerts.
And it's also taking that chance of people coming, of course, maybe to see the main performance, but also to give others the chance beforehand to show their play and to get more exposure to also get hurt.
And then I mean, this is how some of the most famous bands have been rising because they have been the previous band for some other at some concert.
And then people are surprised if they hear that they were just the preliminary band at the very concert if you look maybe just a couple of years later.
So even though it's not the same, but this is just what just came to my mind when you have been talking about, could we say it's user sensitivity towards the content that is being displayed?
That's an interesting term, user sensitivity.
I rather talk about acceptance, like sometimes it's acceptable, sometimes not.
And that may vary across people.
Yeah, but I think that that's indeed a good point.
When you mentioned the concert example, like which band is playing there, you give one band exposure, like exposure and have a chance to reach a new audience maybe.
And it's like the decision, which band will it be?
Yeah, so it's a chance for the band that can actually play.
On the other hand, if it's really a bad choice because the for instance shower doesn't fit or its quality is bad, then it's actually going in the wrong direction.
So it's actually not really helping and also not the end, helping the end consumer.
And maybe the main show is also then not perceived as the best.
They like that thinking about, you say it's acceptance, because then it would towards moving to more fairness, but then also comprise predicting user acceptance within certain contexts.
So I like your meditation example when you say, okay, I somehow I'm able to recognize that the user is currently highly acceptable of that kind of variation within the music.
So this is my chance to slide in some music from some underexposed artists that still tailor to what the user wants to listen to, but not maybe the very except artists, because it might have been the artist that has played the meditation music 10 times before.
So yeah, yeah, I think that could be a chance to look into that.
I haven't done research in this field.
And I think that also relates a lot to what's considered in diversity in terms of how diverse should the set of tracks be like what a user wants.
So some want to have a very diverse playlist and others not.
So there's indeed a preference or more open for diversity or willing or wanting that and others not.
And it's typically not a certain number and that's it.
But there's a range of I'm accepting to have new songs in my playlist that I haven't heard before to a certain degree or to how far away can it be from your music taste that you still accept that.
And that's used in research when it's addressing diversity from the user perspective.
And I think it could also be addressed in a similar way when it comes to fairness in the representation from the artist perspective.
And it somehow it could also be a help for creating diversity.
Maybe however, maybe hard to understand from offline data that you might be having, because this might be something that you should rather do in some online settings.
Yes, you definitely need consumers there.
So user studies, online studies, especially when it's so much about subjectivity, because that would also be changing one's pattern to what one was listening to before.
And for that you have to have additional data.
It's not reflected in the data that you already have.
And what impact this intervention has.
So typical way would be going via user study and then online evaluation.
Which brings us to another point.
So thanks for the word evaluation.
I mean, I was about to promote another workshop first, because there has been a workshop also around fairness.
I guess at this year's RecSys conference, it will be the fifth time that this workshop will be held.
Nowadays, it's called the workshop on responsible recommendations and it's a fat rec workshop.
So the fairness, accountability and transparency workshop.
So definitely worth to keep that in mind if you are going to RecSys.
But let's talk about another workshop, because as our loyal listeners will know, and also the people that visit RecSys or attend RecSys virtually, there is not only the main conference, but also very many different workshops going on that have different topics.
And you're actually the co-organizer of the perspectives workshop.
So the workshop on the evaluation of recommender systems.
Can you just tell us a bit about that workshop, what its purpose is and what we are going to expect there or what your intention is with that workshop?
Yeah, yeah, it's we are in the second edition now.
Yeah, last year was the first one was a half day.
This year we have a full day.
It's called the perspectives on evaluation of recommender systems, because we need to take different perspectives to get the full picture from the evaluation and not only zoom in one aspect and ignore what else is happening.
And then we don't know a lot about the recommenders.
So that's one perspective of it on the perspectives.
And the second perspective is that we as researchers, we come from academia or industry, some are first year PhD researchers, others are in the research business for 30 years, we have different perspectives and different resources to deal with evaluation.
So we have to take all the different perspectives into account.
And what is the purpose of the workshop?
We want to bring all those things together, but also move forward like to make improvements because we see that some like there's lots of offline evaluation for lots of different reasons.
But in comparison, not so many user studies and even less online evaluations.
And the question is, for instance, should everybody do online evaluation?
Or is it only relevant for certain topics?
Or should only industry partners do that and academia should leave their hands from that or not or why?
And also to make the results across different projects comparable.
And if everybody does something completely different and it's not related to each other, then we're not moving forward as a community.
And if we don't do that as a community, then that's indeed problematic.
And that was more or less the starting point for this workshop to happen.
And last year, there was lots of discussion really focused on discussion a lot.
There were papers presented and we also discussed those.
But it was really like, what do we need?
Where we want to go?
How can we achieve that?
That was the main contribution in the workshop and was very inspiring.
And at the same time, we had to acknowledge, yeah, we discussed those things, but we are not there yet.
So this discussion needs to continue.
So I'm really looking forward to lots of papers and lots of presentations, lots of discussion.
The call for papers is already out for a special issue in the new transactions on recommender systems journal, also an evaluation.
Of course, the different perspectives are also welcome.
I guess as one part of the workshop, Tither, there was also the question post about if there is a golden standard for the evaluation of recommender systems.
So is there a golden standard, but just researchers and practitioners are not yet fully adhering to it or isn't there and shouldn't there be a golden standard or what is your take on this?
My personal take is there's no golden standard that would apply for everything.
We just treat some things as if it would be the golden standard for everything.
And then still we do something which is maybe not the golden standard, but it's easier to do because we have data and computational power.
I think we really have to delve into what do we really want to find out to have a clear goal, which direction should it go?
What do we expect and evaluate for that?
And then also look into other trade offs somewhere else, because if we improve accuracy, great.
But if in the end it's always the same item recommended to everyone, it's maybe not the best idea unless that was also a goal.
At the same time, if you have high coverage, but every user would get something that they're not interested in, that would also not be a good thing.
And we already have lots of different metrics, but it's not happening so often that all like the wide spectrum of metrics is considered within one study, sometimes for good reason and sometimes maybe for not so good reason.
I guess of that general criticism that has been rising over the past years, which is also healthy criticism that says, okay, there's too much evaluation only focused on the retrieval accuracy because it might be too easy or there might be just data sets that only allow you or basically bias you towards only doing that accuracy evaluation in terms of precision at K, recall at K, N, D, C, G, M, R, R and all those.
If we only take this picture with regards to retrieval accuracy, then even in that setting we see problems there.
I mean, there was at best paper in 2019 at the RecSys, which posts the questions with, we are really doing a lot of progress.
I mean, it was also about deep learning for recommender systems, but there we have also seen that sometimes the performance of your approach also depends heavily on how you like to perform the evaluation or how strong or elaborate you want to make your baselines.
And if you don't put in too much effort into creating competitive baselines, then of course your approach will stand out nicely compared to them.
So if we would even say that this dimension of retrieval accuracy is a valid one, then what could be a golden standard in that setting?
That accuracy is valid and we need it.
Like without accuracy, I don't know where we would go.
So definitely it's just not the entire picture.
That's my stance on that.
But you were asking for the golden standard.
I don't have a golden standard for you.
There won't be a golden standard, I think.
What I really think in terms of the story you told before, like, are we really making progress?
And I think that's especially an academia thing that we try to solve a problem that we just encountered and we are interested in.
But sometimes the question is, does that matter in practice?
If we achieve this goal, does this make any difference?
Or we easily say, yes, it matters.
Of course.
Maybe we post the question, we just say, yes, of course, but we don't really know.
So it's a lot of assumptions.
And especially as with recommandors, it's all about humans that get recommendations at some point.
And the perception is often very different to what objective measures would expect.
And that somehow brings me back to the fairness thing.
If you ask people if something's fair or not, whether recommendation is fair, if you would ask this question.
Maybe I wouldn't be able to answer that.
And probably lots of users also don't know that.
And then you could say you improved something concerning fairness, but people don't like it.
But maybe they just don't see it.
But if you would label it, that's in the carousel, like one way of the fairest ever recommendations, if that would be possible, maybe people would perceive it differently and then like everything or hate everything to exaggerate.
And we need to take this into account and we can only do that if we include people.
Yeah, there I guess we always want to show what has changed.
And I guess we are all somewhat inclined towards showing this on a quantitative basis.
And there it's nice to use those commonly known and working solutions, even though we could criticize them individually.
So you always basically want to show that you have improved some certain precision or some certain MRR or in DCG by a certain amount.
And for different things that might matter or might have an impact in reality, this is just a bit harder to do.
So for example, let's say, I mean, talking about recommenders in a business setting and we have a business that is focusing to use a recommender to achieve customer retention or to increase revenue or profit or something like that.
This is basically no data that we are having access to.
So it's hard to measure.
So I guess there are two dimensions to this problem.
So the one is you don't have the data for this and then we fall back to what we can evaluate just in the end to say that this is not impacting or just doesn't tell us whether this is having an impact.
And the other one is the general problems that we are having a disconnect because the retention or the sales or something like that is really the final goal or one of the final goals downstream, which tells you, for example, the business goal and then it goes back business goal.
Then you have some online metric, offline metric.
And in the very beginning, you are having some loss you use for your recommender.
So about these two directions.
So the problem of the data and the problem of the disconnect, what are your takes on this?
Well, the problem is the data is it's just some people don't have access to the data and other people have access to the data.
In this case, it's industry like in their own company, they hopefully have access to their data.
And please do it and make it consider in the in the evolution.
And that's also one of the things why we considered for the workshop.
Well, maybe different people in academia and people in industry have to do different things or have different roles on evolution.
And for instance, in like in the fairness field, I have the feeling I want to show where problems are and push people in industry to integrate that.
Because maybe the incentive to start that from industry perspective is different.
So I see a special role here as a research in academia.
There's some some point of really miss here, because okay, we have open data sets and we have access to data.
And then there's something that's done online.
And in the very moment data is collected.
But we also have lots of possibilities in between if we do user studies, and there's a wide range of user studies you could do.
It's also quantitative, or it can also be quantitative.
And if you have substantial sample, that's also representative, you can do a lot of things.
And it's just it takes effort.
And it's not that you like first year of study and you know how to do the perfect user experiment.
Of course, you don't.
It's also a skill that we have, like the skill that we need to develop, but it's a useful one and it will hopefully pay off in the in the end.
But of course, if you have an open data set, the data is already available compared to you have to invest several weeks or months to get the data.
Of course, there is short step or longer step.
But if it pays off in the end, and paying off, I mean, not in terms of money is to find something out that you can use in the end.
Okay, so to get you correctly, you're calling for more user studies to be performed because they allow also people which are not in industry and which need to take care of certain data not being published.
So they allow also those people that don't have access to this data to create the data themselves as a result of user studies and have this as something where I could measure much more than just if I'm doing very good at my very first position of recommendations, but also to use it to question people about what their perceived diversity of recommendations were and how good the discovery experience was.
Yes, yeah, I wouldn't say okay, we need more user studies and it's only about more, they also have to be good because if it's a bad study, then it's useless.
But it's also bad offline evaluation is useless.
Yeah, that's with everything.
But as we deal so much with people, we have to involve people at some point to also to check back if our assumptions that we use in the computational approach make sense and it's reflecting how users stick and this again will inform how to use the user study.
So I really consider that important to do user studies and maybe we come closer to the golden standard like ideally it's a combination.
There might be definitely research questions where you don't need to combine or it doesn't make sense.
But I'm convinced there are lots of research questions where it makes sense to combine lots of different perspectives again.
And also if user study offline evaluation, that's two different perspectives or including a wider set of metrics, considering different domains that's already broadening our view on what's going on.
Yeah, yeah.
So it's a combination.
There's not a single answer.
If there is a lesson that I'm going to make as part of this episode, there is not an easy answer or a one rule fits all answer to all the things.
But that's how life is.
It's sometimes more complex as you want to boil it down to some simple rules.
There's still work to do.
Yeah, we talked about lots of challenges so far.
So about fairness, especially in music recommender systems, about the challenge of proper evaluation.
What other challenges do you see for the field of recommender systems?
I just want to possibly an idea or a push an idea on that.
Yes, go ahead.
Like it's a while already we talk about context of our recommender.
And I feel that when we talk about context, we talk about different things of context.
Again, lots of different viewpoints.
And especially as I came to recommender systems from the context of our computing field, although we use the same definition of context, and in this definition, we cite that a lot, but we have different takes on it, what we really consider.
And so what is context?
What can we include in our systems?
And my impression is that especially in the context of our computing field, lots of sensors are used like really devices to get additional data, additional information about a specific situation about the context that a person is in or the device is in.
And in comparison, how we at the moment do it in the recommender systems field, when we include context, we don't exploit the full potential.
I think for various reasons, like there's no data set that includes all the different aspects.
So we don't have it.
We can't use it.
That's a very simple explanation for why it's not happening.
For other things, it's also a privacy issue coming into play.
Because if you track a person with lots of different sensors, of course, you run into a privacy issue.
So probably better not doing it, or yeah, you have to do it in a way that's okay, privacy wise.
So these things come together, but especially as recommender systems are for years now used a lot, like consumed on a smartphone or a mobile device.
And those smartphones are equipped with so many different sensors already.
Maybe it's at the point of time where we could try to think about what can we collect, what is okay, and then introduce it into our recommenders.
Make use of the accelerometer of your smartphone when listening to music.
If it's useful, but if we combine it with sports, it's very useful.
Yeah, yeah, I see your point.
Definitely.
So to do this kind of more smoothly or implicitly, then wait for the user to explicitly offer the signal.
Even there, we are having basically the trade off.
You need content, definitely.
But if you just tell the user, I want to use this data and you don't say why, some might indeed accept that, but I think that's not okay to do it in such a way.
But if there's a good reason and it's not revealing, or it also depends on where this data goes.
So if it still remains on your device only, and it's not transferred anywhere, that's also a possibility to make it in a privacy sensitive way.
Yeah, definitely.
Even though we might be missing the proper training data then, or additional training data for retraining.
Yeah, lots of different challenges still there.
So it's a challenge that could be addressed.
Humor wise, please address it.
Of course, it has to be done in a sensible way and in a way that makes sense in the way that it has impact in the end.
So if it's just lots of different approaches additionally and lots of more data, but nothing will change in the output, then it would be useless.
But at the moment, I think we don't know as a community.
I actually like the idea and it again, is a good point for there is still a lot of work to do in the field.
And this might be another topic that shows that there are different new ideas constantly popping up that might need to be addressed in the future.
Yeah, I see there's opportunities.
We don't have to address those, but I think there are opportunities.
At least worth exploring.
Indeed.
Cool.
Okay.
Also, one question and you might going to expect this one as part of my three wrapping up questions always is if you think about a recommender that your results you're going to use as a user or what would be the recommender or what is some kind of personalization systems that you really enjoy as a user?
If there is any.
There is, I think there's nothing I really enjoy as a user.
Because then I start thinking, why did it recommend to me?
What did they use?
Which data did I have from?
Or why isn't it any better?
Or why is it so good?
So it's triggering me too much.
It's triggering your research mindset too much.
It's really hard, but I think that comes with the special role.
So you are never falling down any rabbit hole because you're basically interrupting it by your research mindset that just basically tries to tear the things apart.
I don't want to tear it apart, but it's yeah, it's I can't stop my thought process.
What's going on behind that?
Okay.
If you think about, I mean, you have been recommended, I still think a couple of times in this podcast already.
So it was really a pleasure to have you on the show finally.
And maybe we'll be having a talk again in the future.
But which other person are you thinking of or are you maybe having in mind that you would like to see on RECSPERTS?
I want to see Iva Tangela.
I'm working with her and she's doing lots of more different things.
And I think it would be really cool to hear her insights.
Perfect.
Then I will also put her on my list.
So I expect my invitation.
Christine, many thanks for you to taking part in this and also sharing your research, your thoughts and for your contributions in the RecSys community.
Will people that are listening to this show also see you at RecSys this year in Seattle I hope so.
I hope so.
So I'm definitely there, like at least virtually, but I'm strongly planning to go there.
But we don't know how the world situation will look like.
So I'm monitoring this and included in my decision making.
Let's hope for the best.
And then we will meet again, hopefully in person at this year's RecSys in Seattle by the end of September, because this is also at least my intention.
But as you already mentioned, you never know what's going to happen.
But as I said, let's hope for the best.
Yeah, let's hope for the best.
And thanks again for the invitation.
It was really a pleasure talking about these topics with you.
Thanks.
So then have a nice day and see you.
Goodbye.
Goodbye.
Bye.
Thank you so much for listening to this episode of RECSPERTSs, recommender systems experts, the podcast that brings you the experts and recommender systems.
If you enjoy this podcast, please subscribe to it on your favorite podcast player and please share it with anybody you think might benefit from it.
Please also leave a review on pod shazer.
And last but not least, if you have questions, a recommendation for an interesting expert you want to have in my show or any other suggestions, drop me a message on Twitter or send me an email to Marcel at RECSPERTSs.com.
Thank you again for listening and sharing and make sure not to miss the next episode because people who listen to this also listen to the next episode.
See you.
Will you bye and good day.
End End End End End
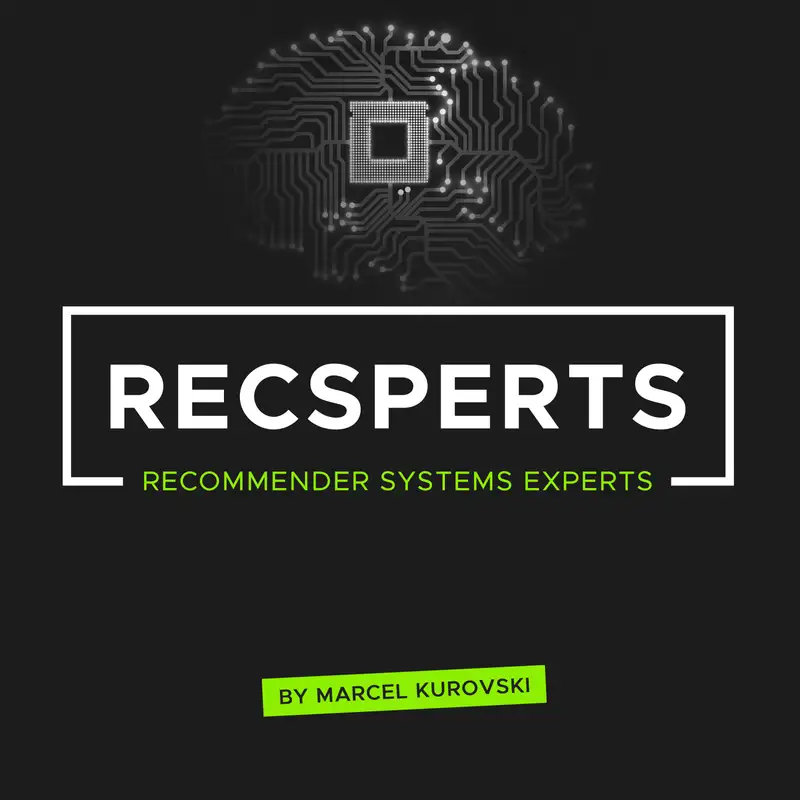