#21: User-Centric Evaluation and Interactive Recommender Systems with Martijn Willemsen
Note: This transcript has been generated automatically using OpenAI's whisper and may contain inaccuracies or errors. We recommend listening to the audio for a better understanding of the content. Please feel free to reach out if you spot any corrections that need to be made. Thank you for your understanding.
In the RecSys domain, everything comes together actually for me because it's about data, which I like.
It's about trying to predict preferences, which I have a bit of knowledge about.
And it's not just about algorithms, it's also always about the user.
I think you always try to predict preferences of a user, so you really have to understand the user well in our field.
The user research actually allows you to interpret your data and then you can start optimizing for that.
But that means that you also have different types of users that you're serving in different needs and that have different goals.
So when you start developing some product, you definitely have to start with user needs.
And that's what I also train my students in my course.
So you're building a recommender system and the interaction with that recommender system is crucial in understanding your user.
Implicit data is very hard to interpret.
You can have a click on something and that can mean that a person is interested, but it could also mean that that person is just looking around and maybe even lost and confused.
In a lot of our studies we actually find that experts are typically not so happy with recommendations because they know very well what they like.
It's more easy to be wrong with an expert.
Hello and welcome to this new episode of Rexburts.
I have to admit it has been a while, but now we are back on track and there is already a very great lineup of upcoming guests for this year.
But as always, I'm welcoming guests from the academic and from the industrial side of recommender systems.
People who do research, who apply recommender systems and people who contribute to this field and the community.
For this year we have already a great lineup of Rexburts from Matter, BBC and from Pinterest.
And I'm looking forward to many more.
But as a starting point for 2024 I'm very excited to welcome another luminary of the field who is with me today and no one else than Professor Martijn Willemsen.
Hello, welcome to the show Martin.
Oh, great to be here.
Thanks.
Yeah, it's nice and I guess it's quite a while since I've already reached out to you and asked if you want to attend one of my episodes and talk about your research, about your experience, about your contributions to the field.
And it took me a while to get back on that.
I'm very happy to have you on the show today.
Before I hand over to you, Martin, let me provide a bit of background.
So Professor Martijn Willemsen is an associate professor at the Geronimos Data Academy and Eindhoven University of Technology.
He is also a program director there and obtained his PhD in psychology from the Eindhoven University of Technology, but not only his PhD he obtained from there, but also his master's degree in electrical engineering.
So quite a broad scale of different competencies and fields and I guess quite a nice match of things to combine in his research and in his work today as a professor.
He has been contributing to the field in many ways with contributions to various journals, to the RecSys conference, of course, to conferences like UMAP and CHI.
And now you actually have to help me.
I guess when RecSys took place in Amsterdam, I'm very much sure that you have also helped some organizational, some committee membership there.
Is that right?
I was the organizer, so yes.
He was the organizer.
One of the key organizers, right?
So RecSys 21 was the one that actually was the first hybrid one.
It was in 21, so it was in the midst of COVID.
In a small time we actually were able to have a physical conference, but of course not all the people from the field could join.
For example, at the time Europe didn't allow people from the US easily to fly into Europe, for example.
So we had the first hybrid conference in the birth of Berlach in Amsterdam.
And I organized that together with Marta Larson and Umberto Corona.
So these three were the organizers for the RecSys in 2021, which took place in Amsterdam.
And it was really a nice building, a very nicely picked place.
Of course, it was a bit unfortunate due to the circumstances that not as many people had been there as in the years before, but I guess this is nothing that we had under our control.
So this was something due to, as you already said, external circumstances.
Nevertheless, it was a great place and also nice, at least for the people in Europe who had not traveled that far, as they obviously also don't have to in this year, where RecSys is going to take place in Bari in Italy.
But this is not the only thing that you have been doing.
You have been doing a lot of research in RecSys and this will be also our main topic for today.
So we are going to talk about decision psychology, about your user-centric evaluation framework, about interactive recommender systems.
So lots of interesting topics, but I guess you are the better person to say more and introduce yourself.
So, Martein, please tell us more about yourself and your journey in recommender systems so far.
Okay, thanks, Marcel.
So yeah, it was quite a journey.
And as you already told the public, it's basically, I have a very multidisciplinary background.
So I started electrical engineering, but became quite bored of the very technical part of it quite soon.
So I did another master in technology and society, as it was called at the time.
And there I got very much interested in the psychology of decision making, how people make decisions, how people make judgments.
That really intrigued me and that made me choose a PhD program at our university, at a professor who was a real decision making researcher.
Yeah, I basically turned myself into a psychologist during my PhD, which was really fun.
And my PhD was actually about how to measure preferences and the fact that preferences are really hard to measure and that they are very unstable and that there are different ways of measuring them.
You can measure them by asking people a choice, but you can also ask them by asking judgment like a scale, or you can ask them to what we call matching, where you ask them actually, okay, you have two options here.
And what of this option B should change such that A and B become equally attractive?
So there's different ways of measuring preferences and you get very different results in terms of what people like.
Do they like A or B better?
And a simple change of question collects the refer to preference, you call them preference reversals.
And that part of the fields intrigued me a lot and it still does.
And it very much applies to the recommended systems field.
I think we're going to talk more about that later today.
So that was my PhD, but quite soon I was able to get a Dutch Feeney grant, which is from the Dutch Natural Science Foundation.
And that allowed me to actually do a postdoc in New York with Eric Johnson.
He's a famous decision making researcher, actually wrote a book, The Elements of Choice, which I would highly recommend to any listener.
We should put that, I think, in the notes.
And I did a lot of process tracing with him.
I built a tool to process decision making processes online.
This was 2002.
The Internet Rule just started.
And we built a tool to actually study how people make decisions online.
And that tool actually generated a lot of data and I like data.
So that actually spurred my interest more into the mathematics and the statistics around data.
Bouncing back to the old electrical engineer?
Yeah, maybe.
At least a little bit.
At least the mathematics.
That's very strong in that field.
And I become very data driven.
And then across the years, I started to do more of that.
And then data science became a hot thing around 2010, 2011.
It started.
At the same time, the RecSys community also was growing and some people actually asked me to join a European project called My Media, which was a European FP7 project.
And they asked me to join that because of my decision making expertise.
In the RecSys domain, everything comes together actually for me.
Because it's about data, which I like.
It's about trying to predict preferences, which I have a bit of knowledge about.
And it's not just about algorithms.
It's also always about the user.
I think you always try to predict preferences of a user.
So you really have to understand the user well in our field.
And as soon as I entered that field and I saw the conference and it was very algorithmically driven, but there were really few people that were doing interesting user studies and having interesting ideas about the psychology behind it.
And they also referred to the same literature I came from.
So there was even a workshop on that topic.
So I was like, okay, this is really interesting.
So yeah, that's when I actually joined the field.
This was around 2009 or 10.
And since then, yeah, we developed several things.
So I think the evaluation framework we're going to discuss later as well.
It was developed in that project because we actually had to evaluate a bunch of recommender algorithms with partners in that project.
And then Bart Keneideberg and I developed that framework to actually do a good job at measuring that.
Then we published that and it took off quite well.
And since then, I've been doing a lot of psychology oriented user centric evaluation studies in recommender systems.
And I joined the conference more and more and became more active, became a senior PC member.
And at some point, people ask you, would you like to help organizing the next RecSys?
Not knowing that there would be something happening like COVID.
But we managed in the end and I really still like the field because of its multidisciplarity.
What I also learned is basically it was not just RecSys because there's a lot of other conferences like RecSys like IUI, CHI, UMAP, that also do a lot of user oriented stuff.
And because of RecSys, I got to know these fields as well and then actually found that this is really the sweet spot of my own interest, how to basically develop smart nowadays, we would call them AI technologies or whatever, interactive technologies that interact with humans and how you can evaluate those and how you can make sure that they actually align to users mental models and users needs.
All my research is basically oriented around that.
I think that sort of sums it up.
This is definitely a very informative and well crafted overview, how you joined the field and how you took advantage of all the different directions that you have been going through and collecting experience in from the electrical engineering from the rather purely psychological side and then becoming data driven.
And finally, kind of merging all of these aspects in the RecSys field.
So must be quite hard for you to choose when you have an idea that you want to elaborate on whether you are going to submit it to RecSys or to one of the other many conferences there are for these topics.
Yeah, maybe but so the thing is these are all different deadlines.
So it also depends a bit on when you do the study and what is the right deadline to still be able to submit a paper.
I am also doing a lot of research on explainable AI and trust in AI.
For example, that is something that fits much better in IUI as a conference.
So then it's easy choice.
But indeed RecSys papers go can go anywhere.
I prefer to publish them in the RecSys conference, of course.
Yeah, but I also have a few that are in other conferences.
But I think it's sort of it's actually interesting that you say this because you see a lot of RecSys papers outside of the RecSys conference and especially if it's not so much about algorithms or it's more about users.
But for example, also the SIG IR conference has a lot of RecSys work.
Sometimes it's a bit of a pity that some of these papers don't get into RecSys because it would be nice to have all these papers there.
It's the main conference.
But I can also imagine that people come from different fields and that deadlines fit better with different conferences.
So sorry that the deadlines fit better with their process or that they it's their own main conference.
No, I still remember the RecSys conference in Amsterdam quite vividly.
And even though it was in the midst of the pandemic, it was still a great conference and also a nice occasion to get a bit outside.
And I mean, we were also able to do a small karaoke session if one can say so here.
Always part of RecSys.
Unfortunately, I haven't been there when it took place in Singapore, but I'm looking forward to attend this year in Bari, which is a bit closer to say.
But yeah, then of course, also I'm looking forward to some great karaoke sessions again.
But something that I found interesting is what you have just said about the community.
And it felt like back then in 2009, 2010, when you were attending for the first time, if I'm right, that it felt like, oh, okay, there's a large focus on algorithms, but less on really the psychological aspects.
And I guess it wouldn't be fair to say it's more of the softer aspects, but it's then I would say more of the underrated or ignored aspects, because sometimes it's just easier to take a bunch of data, apply some algorithm on top of it and then say, okay, this is my result.
And I have beaten this and that compared to saying, okay, how this is going to really change people's lives or enables them to follow their needs, goals, whatever.
So do you feel this has changed or in which kind has it changed over the course of the almost 15 years?
It has changed, but it's more like waves.
So RecSys is like, I think we get to the 18th conference, right?
But anyway, so I think it started 2007, 2006, 2007.
It started off as a very user centric field actually, because at the time there wasn't much data and it was really about how to recommend to people and you had small studies and the user aspect were obvious at the time because people were still figuring out how this works.
Until the 2010, 2011, basically all these new algorithms came about, the matrix factorization that people used to win the Netflix prize, right?
Basically the Netflix prize itself that actually wanted people to improve the algorithm by 10% is reflecting the very nature of RecSys.
Like we need to improve our accuracy with a little bit and then be very happy.
So yes, in that period, people were very focused on that, but that wasn't the entire community and the community as a whole always been very open to also to user aspects.
Then I think around 2016, 2017, 18, the user aspects are very central actually.
I always use one slide of the 2018 conference where that showed how many user studies were actually accepted for that conference.
I use that in my classes to show the students, it's not about algorithms yet.
It's also about users.
But since then, I think with the deep learning coming around the corner and more of the RecSys community focusing on a lot of clickstream data, implicit data that you can get from all these systems that all become also more digital, right?
The data is now coming from streaming servers or from a website or from some sort of app.
So you can collect a lot of implicit data from a user using your app.
And we assume all sorts of things behind the data and we optimize our algorithm on that.
And that's different from the old systems that used ratings.
And you saw the field moving towards that implicit feedback data because it's easier to acquire.
It's also much richer and much closer to people's actual behavior.
So it's much easier to optimize.
So this field has moved, at least part of the field has very much moved to building recommender systems that try to optimize sort of what to show to a person at a particular moment.
And with all the data, you can do that quite well.
You can use complex algorithms like deep learning to do that.
But that's only part of the puzzle, I think, because we are ignoring a lot of things.
By doing that.
But that is actually what happened, I think, in the last five years, five, six years.
RecSys was actually quite late in embracing deep learning.
It was like, I think, 2017, 2018.
I guess it was 2017 when the first workshop on deep learning for RecSys took place in Como, I guess.
So, for example, if you have a clothing website, right, you're selling clothes, you can use deep learning to turn the images into the right features that you can then build your recommender system on.
So deep learning was more instrumental to feature algorithms with the right information.
At least that was my understanding at the time.
And then quite soon, also, people found out that deep learning is great for optimizing your algorithms.
That's part of the field.
I'm not really working in that part, as you can imagine.
But you see that has become very strong in the field itself right now.
But still, there's a lot of other approaches and interests.
And we also have a lot of people working on fairness, for example, right?
And still a lot of people doing user studies.
So it's still a very nice and multidisciplinary field, I think.
Yeah.
And you have already been providing me with my talking points very well so far.
Talking about fairness, talking about waves, which I guess brings us to the first main topic for today.
But actually, before going into that one, Martijn, you were very right.
Exactly on point.
We are approaching the 18th conference this year.
And the first one took place in 2007, actually, in Minnesota.
So yeah, so much as a background.
Yeah, the point that you are bringing up about the waves and when user studies gained more attraction brings us also to, I would say, our first topic for today, where you teamed up with another guest that I had on my podcast quite recently, Michael Extrand.
And together with him, you wrote a paper in 2016 that was called, Behaviorism is Not Enough, Better Recommendations Through Listening to Users.
In that paper, which was eight years ago, but which has not lost in any relevance for us today, you are even going back two years further, which actually brings us to Netflix again, by actually citing the chief product officer of Netflix, Neil Hunt, who back then was quoted from a keynote that he gave at RecSys 2014, saying that Netflix metrics cannot distinguish between an enriched life and addiction, which is quite a bold statement.
That was already kind of the hook for that very engaging talk that Michael Extrand gave when presenting that paper, in which he claimed, listen to your users, at least sometimes.
And kind of the starting point of something that claimed by just looking at behaviorism, so at, let's say, mostly implicit feedback data, we are missing out on other things.
I have to be honest, when we were doing our introductory talk, I wasn't actually aware of the paper, so I read it, I've seen the talk and I have to admit it was great and interesting.
Can you walk us through what this paper was about?
Yeah, there are several claims in the paper. I'm not sure I'm going to address all of them in detail, but let's first start with this behaviorism point, right? So the title of behaviorism is not enough, and it was a reference to the very early paper by McNeigh, which was labeled, accuracy is not enough, from 2006, in which the group lens group from Minnesota was already making the point that we should look a bit further than just looking at accuracy. And Michael and I saw sort of the growth in people just looking at implicit data only and looking at click streams and taking that point of Neil Hunt, but also, yeah, in general, the fact is that implicit data is very hard to interpret. You can have a click on something and that can mean that a person is interested, but it could also mean that that person is just looking around and maybe even lost and confused. And actually, the with the framework that we developed, we already found this out in 2010, there was a, we did a study evaluating one of our recommender systems in this My Media project, and we found actually that when we gave people actual recommendations rather than random recommendations, they were clicking less, they were less engaged, they took less time using our system, and we were a bit worried that our system was actually failing. But we also had some subjective metrics, we asked for satisfaction and quality and stuff like that. And we found actually that they liked the actual recommendations more than the random recommendations. We dig a bit deeper, and then we found actually that these clicks are negatively correlated with satisfaction and quality. And then digging again, a bit deeper, we found actually that if you take the time that people watch the, it was a video recommender, whether people watch the movie from beginning to end as a metric, that was positively related to getting recommendations. So the people that got recommendations, they found movies that were very relevant, they watched them beginning to end, they clicked away and stopped using the system because they fulfilled their goal. And the people that got the random recommendation, they were clicking around a lot to find any movie that would actually be somewhat nice for them. So they were lost. So the clicking was not engagement, it was just confusion. So yeah, what is that click meaning? A false positive in the way you interpret it?
Yeah, exactly. So that interpretation is really hard. So that is one aspect that we want to discuss in the paper. But I think the paper goes deeper than that. The paper is really about, in the end, you have a particular goal that you want to achieve with recommendations. And if you keep people reinforcing what they're currently doing by just, yeah, if you're watching Netflix and you like a particular show, you get the next one and the next one, and you keep on clicking and you're liking that stuff, or YouTube would be another example. Then yes, sure, the data shows that people take your recommendations and that they keep on clicking and that they really like it. When you say by a goal that you follow, then you mean with you the platform provider or kind of the entity that runs the recommender system. I think the user has a goal. And of course, the provider also has a goal. The provider just wants you to keep paying for your service for the service, right? And they found out that if they keep sort of optimizing these clicks, then yeah, the people watch a lot and they stay with the service. But our question was, is this really adhering to their own goals, right? So if your goal is to watch a bunch of funny movies, or is your goal actually to really enjoy and maybe even get a bit deeper and get a bit more insight in particular problems by watching a documentary or whatever, right? You probably have different goals when using those systems. I think just looking at implicit data is not bringing you these goals. So one of the arguments that we make in the papers, you should listen to your user, which means that you should also go to your users and ask them you can use focus groups, or you can use surveys or whatever, sort of more subjective ways of measuring their needs and their preferences and their goals.
That's of course, a bit different when you talk about a movie or music recommendation versus helping people to live a more healthy life or save some energy. And especially in those domains, people will use those systems as well as to sort of to improve themselves, right? And then they have a particular goal. And then the whole notion of basically recommending what you're currently like is suboptimal because recommended systems just base their recommendations on your historical data. So they try to predict what you liked before. But if I want to live a more healthy life, and I eat a lot of hamburgers, yeah, you're going to recommend me more hamburgers because that's what you did. But you probably need something else. You have a different goal, which means you might also need a different algorithm. But the first step, of course, is understanding what needs those users have, what goals they have, and then make a system that actually help people to achieve those goals. So that's one of the points, there's more points, and you actually read it recently. So you might remember better than I do.
No, something that I would like to dive into at that point, since it might also elicit some of the conflicts which exist between user and platform goals. Keep in mind the user as the recipient of recommendations, and the entity that is consuming recommendations, and the platform that kinds of, yeah, recommends to say, then one could easily make the claim that their interests, their goals are not always explicit and clear, even to the entities themselves. And second, not well aligned. And in that sense, what I like also about the paper is that you, when starting with the why, why you should listen to your users, you make these two directions, and to say, okay, because of pragmatic reasons, but also because of philosophical or ethical reasons, because you should listen to users, you should respect their wants and their goals.
But maybe staying with the first one, so the pragmatic reasons for it, which somehow assume to say, okay, if you do so, if you listen to your users, you will also benefit as a platform.
And this is something that I would like to question. Let's say I'm a food chain, and have my customers shopping on my platform, and have just, let's say, a higher margin with recommending or with products that are rather unhealthy. Which means that if you as a platform want to grow profitability, you have rather interest in recommending, let's say, the high profit margin food to your users, which doesn't necessarily mean that users, if they consume unhealthy food, will stay for lesser time. So they could still even stay as long as they want. So within that example, so do you think that unaligned goals could be a problem? You're adding something to the equation, because the company has a goal to satisfy the user, right? It's trying to, if you have a recommender system, it's building a user model, and you try to predict the best thing for that user. Our algorithms are trying to optimize in such a way that you get recommended to options that the algorithm thinks are the best predictions for you, right? So and now you're adding the thing to the equation that the company, of course, also has other goals, right? They want to make profit, and they could actually make a trade off between, okay, this fits with your preferences, but this actually makes more money for us. So they could actually trade off these two things, and then it's a user goal versus a company goal, which is also a bit of a multi-stakeholder problem, which is another topic which I'm not an expert on, so there's definitely a few other people you can interview about them if you didn't do this already. But this is more about satisfying the user, but also helping the user, right? So at some point, if you keep recommending stuff to your user, that will make him feel that he's sort of not living very healthy, at some point he will probably feel bad about that and might stop using your service, right? I think that's one of the points. And for example, I think there's a lot of companies, and this includes Netflix and Spotify, for example, that do a lot of user research. Actually, when we came into the RecSys field in 2010, with the first workshop on user-centric evaluation, Netflix gave a keynote on how actually they did a lot of user research, and Spotify has their own research teams doing a lot of this.
So I'm not saying that they're not listening to users at all. I think Spotify has some great examples on their website actually showing that listening to users actually helped them a lot to understand, for example, their Discover Weekly. The Discover Weekly or Spotify is really the core of their recommender system, I would say. And they had a hard time understanding the usage data of that and to evaluate whether actually people liked Discover Weekly. Because some people actually just headed on for hours and other people just clicked on the first song they heard and went to the song and went out of Discover Weekly. And they actually found using surveys and interviews that are just four or five different patterns of behavior that have very different implicit data behind them. And then the user research actually allows you to interpret your data, and then you can start optimizing for that. But that means that you also have different types of users that you're serving in different needs and that have different goals, right? Some people use this as a Discover Weekly to discover new music. So as soon as they find music, they go, oh, this is really interesting. Let's go there. And they go to the album and you lost them for Discover Weekly, but they actually discovered something new and that was their goal. Other is like, okay, it's a recommender system. So it gives me a nice playlist. I turn it on and while working, I have like three hours of nice music. And that's a very different goal. But this is actually a very great point that well resonates with me right now. The reason why you should listen to your users is not only to understand their needs and goals to serve better recommendations in a more explicit feedback channels. So by performing user studies or something like that, which is not as scalable and as abundant as implicit signals are. But, and this is something that I found very important, that it basically enables you to take your behavioral implicit data to really make sense of it. Because sometimes as a data scientist, as a product manager, you basically have these assumptions. I guess they are not generally or necessarily wrong to say that, oh, there is a conversion, a user purchased something. So it's likely that they like it. But maybe there is a more complicated signal that is maybe a composition of various signals in a specific sequential manner, or also across different parts of your application that then tells you if you take this, this is a much stronger or a much more reliable signal. So basically also helps you a bit more to engineer your data that you are training your algorithms on.
Yeah. Okay. So yeah, that and that. So that was also I think the point of that movie recommender example I gave earlier. And that is that is more about how to evaluate these systems. And our framework is one way to do it. And there's actually a pretty strong community on evaluation, a bunch of workshops that have been going around there and some dachsthuls, where I'm actually going to be next one in May, where I'll go. I'm not sure whether you know what a dachstul is, but I saw a couple of pictures from various people going there. Yeah.
So the dachstul is a little castle in Germany, in Sarland, which is like in the middle of nowhere.
And you're basically locked up in this castle with a bunch of other scientists. And you they do provide you with internet and a very nice library. And you sit together to do to talk about the new things in the field or develop new ideas. Typically, it's multidisciplinary.
So we have been we had a dachstul in 2018, these people from information retrieval, NLP and Lexus.
And it was all about how do you evaluate those systems. And exactly what you're mentioning now is one of the insights. So what is your, what sort of data reflects what sort of goal and you have so low level, if you're a data scientist, you're building an algorithm and you try to optimize that algorithm and your goal is actually to improve accuracy and you choose a few metrics that would be relevant. But behind that accuracy is probably something like, yeah, user retention or take true weight or making some profit in any way. And there might also be user satisfaction.
And it would be great if you could actually connect those measures, but we haven't really gotten far there yet. But I think our that little discussion we had there actually was arguing that we should try to connect all these different evaluation metrics that we have and see, for example, how can we relate some sort of objective metric to people's perceptions of quality or perceptions of diversity or, and that's actually not so hard. I have another paper with Michael actually about on that, but also beyond that. So how does your accuracy measure turns into some sort of retention that later actually tells you, okay, there's not a one to one match probably. Yeah, yeah. It's always kind of which signals or composed signals are mostly or highly aligning with the goals that you are pursuing. Yeah. And now we're back actually to measuring not so much to the goals. So we started it. So I diverged a bit, but let me get back there related, right? Because there's so the also the data scientist has a goal and that user has a goal and that you actually that goal might be different from time to time, right? So I probably have a different goal turning on Netflix when I'm with a bunch of friends and six packs of beer, then then I'm there with my wife and a good bottle of wine, right? Yeah, right. But I mean, the data scientists intent should be the least relevant because I mean, in the end, the data scientists should take care of helping to pursue the user's end or the platform goals. Yeah. Maybe the other way around, but depends on what is the data scientist as a consumer. Yeah, no, I agree. But then this is still a hard question, right? Because how is that metric that is that she's optimizing for as a data scientist connected to in the end to user needs and goal? Which also lives a lot from the clarity and also in a sense, the scalability of expression of those goals and needs, doesn't it? I guess in that paper, I've coming across a term that I liked, which is participatory design. I guess there are two points connected to it first. And this was also, I guess, brought up in the Q&A afterwards, do we even think that users are able to understand their goals and needs, which is kind of a precondition to communicate them? And the second is, how can we create a mechanism that supports them to communicate those needs and goals to us as, let's say, the platform or the entity running the recommender system so that we can help them to achieve those goals? Can you touch a bit on these two aspects? Yeah, the participatory design actually is a way to avoid a lot of problems. So I completely agree that you can ask users, but you not always will get a good answer. That might be CTs already, right? So how do I measure a preference? It's really hard. Now, how do I measure goal even harder? Because a lot of our goals are latent.
The nice thing about participatory design or any other sort of user-centric methodology is that if you do it right, you try to do it within the context of the thing you're trying to achieve.
So by taking the user into the design process, the user will actually also see what you're developing and I think the important thing is that it's very hard for me to understand my goals unless I'm actually engaged in the domain we are talking about. That's the same with preferences. I'm not always sure what I want to watch, but then I get a few recommendations and those recommendations actually trigger things in my mind that help me sort of zoom in onto my preferences and needs at that moment. But I think with goals it's even harder, right? So okay, we might have sort of high level goals, but we all want to live more healthy and be more faced. Most of us. Yeah.
Some people might actually not, right? That's also true. I guess one of the things that you are doing or also doing research in is actually energy saving, which might be a good field. The other one is actually, I guess, somehow health related and something that crosses my mind all the time is actually language learning apps. So many things. Yeah. I think behind the language learning app is some sort of adaptive system that you try to learn something, it tests how well you're doing, and if you're doing well, it makes it more difficult and it takes you to the next step or the next level. And this notion of basically ability, either in language, but it could also be in energy saving or in terms of health. So I think the whole point is that if you want to reach a particular goal, you have to see where you're now. And the goal is like somewhat further or very much further or whatever, right? So a goal means that actually there's something far away that you want to achieve and you want a way to achieve that goal and you need to be helped. And in the behavioral science literature on goal achievement, there are all sorts of models that first assume that you are motivated to actually change something. They typically advise to take small steps to come closer to a particular goal. And in energy saving, we took that as well. We took actually some psychological research on measuring energy saving attitudes more objectively.
So you can ask people, do you want to save energy or do you like to save the environment? And they will say yes, most of them, but it might be better actually in this case to look at their behavior.
So some people have high energy saving abilities because they're actually already doing a lot, right? They turn off the lights, they turn the heating down, their showers are not too long, right? They might actually drive like the car or have solar PV or whatever. It's easy to turn off the lights. It's somewhat harder maybe to buy the energy efficient fridge or washing machine.
It's even harder to put solar PV in your home. And it's very hard to find properly clean energy in Germany to fuel your electric car. Yeah, that's okay. That's a very different discussion.
So there's different levels of difficulty of the items. You can actually monitor how or ask people what they actually are doing and by that estimating their ability. So if I see that you're doing, you are indeed turning off the lights all the time and you turn down your heating a little bit at night to save energy and stuff like that, then my recommendation might be to buy a more energy efficient fridge because that's probably not what you're doing. If you actually already bought this energy efficient fridge, you might actually be the person that can now take the next step to do solar PV. Now from a recommender perspective, so we are now describing users in terms of their ability and items in terms of their difficulty. There's a very simple model behind this, a called the Varsmolo, and that can model basically that relation. And then you can actually do recommendations that help people move forward, to actually help people to achieve a goal in that sense.
So that is one upper realization on this. Now this is going beyond the participatory design we were discussing earlier, but that is a very different approach than our normal recommender systems do because inherent in this model is the notion that you have a particular ability that can increase actually by doing more measures, doing more energy saving measures. And it's not recommending what you already did, it's actually recommending what you should do. And I think in the paper we also argue that we should actually look at recommender models that actually take this into account, this progression that you... And of course not for all, in all cases, maybe it's not that important for movies or music, although you could argue the same for music, right?
You might have a goal to learn a new genre, you did some research on that, or to develop a new taste or whatever. Or if you have actually the goal to participate proficiently in discussions about Star Wars or Star Trek. I mean Star Trek has really some also philosophical touches sometimes, which I really enjoyed when going through that, I guess the last year. So it actually was Star Trek's The Next Generation, but I was kind of astonished of how many also philosophical questions were brought up in that series. So it was nice to see it. Yeah, and this is a nice example, right? Because Star Trek is not for everyone. So there might even be something like this in movies, right? There's aspects to this movie that it's not just entertaining, but it's also educating in a particular way maybe, or getting your interest up in a particular thing. There's probably an ordering of movies that can guide a person that is still watching Die Hard to become a Star Wars lover. Or, right? Or Star Trek lover, depending on which side you are. Just for the record, I didn't mix them up. So we all know that Star Trek is the thing about Darth Vader, right?
How can I sell this to my product managers or whatever and to tell them, hey, before we develop a recommender based on things that are well established in recommender systems application and optimize for some relevancy metrics, shall we not first think about our users, perform user studies and understand really what they want before we start out anything?
You might get cut off, okay, please be pragmatic and please get something delivered quite quickly.
And I'm just trying to think how to strike the balance there. Do you have some advice there or what is your take on that? Yeah, I'm actually even teaching a course that has this UX part into it.
I'm not a UX designer by training. Okay, maybe UX design is about how to design a good interface, but there's more than that. It's actually how to design a system towards users needs. And the participatory design you were mentioning earlier is one way to do it. So that you evolve the user into the design process. But you can also just start with interviews or surveys, or you start with focus groups. But at least you start with a user to develop a product. Now, I think most companies know that I'm teaching in this Uranus Academy of Data Scientists, which is a master in data science in business and entrepreneurship. And we train also our students there to think about the user, right? Because if you if you're building a new business or building a startup, yeah, you good startup actually starts from a very good user need, because then you're probably going to have a nice niche in the market and be successful. So when you start developing some product, you definitely have to start with user needs. And that's what I also train my students in my course, how to do that. And you start with interviews or focus groups to understand what the basic needs are. And then you develop a prototype. And then you actually going to test that prototype.
And one of the things that users are sorry that my students really learn from that is that they always have very nice prototypes developed. And they're really happy about them. And they're really thinking, okay, this is going to this is going to nil it and then users start using it, and they totally dislike it. And they don't understand the whole system and what it's trying to do and why are the why the buttons are like this and not there. And so with user testing, you learn a lot. But I don't think this is actually your question because it's more about because that's typically what the interface designers of your company are probably doing, right. But that's also the point, I think it's not just about in the end, designing a nice user interface that user interface is connected to the system that's behind it. So you're building a recommender system. And the interaction with that recommender system is crucial in understanding your user. Actually, from that interaction, you can learn a lot about user behavior, you can even build a recommender in such a way that you actually learn more about people's preferences by shaping the interaction in a particular way. Then I think, yeah, you will need to do a lot of user studies to figure out what is best. And then I would start indeed with small skill experiments where you try different versions of a system. And you carefully measure people's perceptions and satisfaction or experience with the system and connect that to also the objective data. So our framework that we developed has all these components. And the notion is that you actually do an A-B test, which is common in the industry, right. Normally, if you want to have a new version, you just develop the new version and you test it against the current version, right. You do an A-B test. That's the standard practice in industry. Our approach is actually identical. We also do a test between one version against the other version, but we're not just testing them in terms of what people do, their clicks or purchases or whatever, but we also look how they evaluate these systems. For example, you have now just made it so you listened very carefully to my arguments about making sure that you adhere to user goals. So you somehow figured out an algorithm on your social media platform that would not only just give nice click bait, but also would adhere to some latent goals. You probably have figured out that this user wants to learn more about this or that. And you're going to push him some more messages on that topic. And you built this great algorithm that is actually a goal-based algorithm, whatever. And we're going to test it against the old one. You can implement it and see if people click more, click less, stay longer on the platform for sure. But first, as we already discussed, we don't really know what these measures mean.
Again, if you indeed succeeded in giving that user more relevant content that really adheres to these goals, yeah, two things can happen. That user is going to click a lot and read a lot of content. Or normally that user would be clicking a lot because he was searching for interesting stuff. And now he doesn't need to anymore because all the content is relevant. So you don't know, right? So you want to tie those metrics and what you're doing to some subjective metrics.
And in this particular case, we would take out a small user group, small in terms of the normal numbers that you have as a as a as a recommender company, but not small in terms of normal user studies, because we typically take like hundreds of people in these surveys, like 100 or 200.
You give them the two different versions, the one group gets the one version, the other group gets the other version, then we ask them a lot of questions. So if this is your fancy goal based recommender system, yeah, we might want to ask questions about, do you think the recommender helped you in achieving your desire to read new topics, whatever, right? And some sort of questions that touch upon this goal. But we can also measure things like diversity, perceived diversity, or perceived quality of the recommendations. All those things are perceptions from the user of the system. So how does the user perceives the output of the system, and you hope that your change in the algorithm actually gets true to the user, right? That the user actually perceives that the algorithm is more diverse or more goal oriented or whatever. And then from those perceptions, we expect that if indeed that new change actually resonates with the user, that he is also more or she's also more satisfied or finds the system more effective or whatever measure you want to measure there. So we measure both the perceptions as well as the experience with the system. Okay. And we argue actually that your experience is driven by something you change to the system and how that resonates with your perceptions. And this type of approach really helps also to understand what didn't happen. So suppose you built this new algorithm and then your A-B test, you find no difference. Yeah, then this framework actually allows you to see why, because you actually, yeah, you might actually have improved the goal directedness of your recommenders, but you might actually have also reduced the diversity a lot. And actually, people's satisfaction is driven by both these two things. So people like diversity and they like the stories to be more goal driven or whatever. And one is a positive effect, the other is a negative effect because the diversity goes down. And now my satisfaction is still at the same level because you improve it in one way, but you reduce it in another way. So these perceptions, the drawback of podcasts is you cannot draw on the whiteboard what you want to argue. But these perceptions are in between what you change to the system and how people experience the system. And then also how they behave with the system. Of course, you cannot do all these studies all the time. But I think whenever you're making a major change to your system, for which you also have good reasons, right? So this goal driven example is a nice one, right? So we figured out that users of our social media system really have alternate goals that we're not really serving right now.
We have discovered a way to actually make our algorithm do that. Then, okay, we really want to know actually whether the algorithm is doing what we thought it would be and do subjective metrics allow you to actually say why that happens or doesn't happen. And the nice thing, if it doesn't happen, you actually know why and you can stop doing it or you can fix it.
But it does require you to really understand what are the important underlying factors for that user or for a user in general. So you need a little bit of the psychology behind it as well. But I think there's quite a lot of work out there, not just our work, but there's a lot of user-centric researchers in our field that talk a lot about underlying sort of perceptions and evaluations or experiences that you can measure and that might be relevant in a particular context.
So this such a user study, I think, would be very valuable for any company when they're trying out very new things that might be very promising, but also very hard to measure whether it's actually effective. So I'm not talking about, yeah, if you want to change the color of a button or do a re-ranking, but oh, even that maybe, like you're doing a re-ranking of your algorithm output because of some reason. Yeah, you could actually ask questions about that re-ranking, the perception of that re-ranking and people's satisfaction and to see whether that little thing that you changed, whether that actually resonates. By the way, then, if you also measure the implicit feedback, you measure their clicks and their viewing times or whatever, then you also have the objective metrics that go with this change in behavior. These metrics are then also connected to measures of experience. If you do this once in a while, I hope that you at some point get also a better feeling of what your objective behavioral measures mean. And I'm not saying that you should keep doing this. If you do a good user study like this and you learn a lot from it, you might then take that learnings and then maybe in the future use a different implicit measure because you found actually in your study that that's actually the measure that resonates with satisfaction. So that doesn't only add value for the current experiment, but that it also adds value to properly evaluating future experiment because you better understand how certain objective measures correlate with subjective satisfaction to say. Yeah, so one of our early papers, this was also with Michael Exfrant, was about user perceptions of algorithms. I was visiting the Gooplands group and we're having a discussion that actually, this was the time that we just developed this framework and they were actually discussing that we actually don't even know how people perceive algorithmic output and more importantly, we don't even know that our different algorithms also have different perceptions. So we did a very, actually a very basic simple study where we gave people a bunch of different, we gave them two lists, one generated by, I think one was a matrix factorization, another was a user-user collaborative filtering algorithm and we asked them about their perceptions, the perceptions of novelty, diversity, satisfaction and we found quite some interesting differences there. We found actually that people don't like user-user because it actually gives two novel items. But my point actually here is that we had these subjective metrics, but of course for a lot of subjective metrics you can come up with objective metrics. If you talk about novelty, it's easy to make an objective measure for novelty, but it's the inverse of popularity, right? Same for diversity, we had diversity there as well and there's all sorts of objective diversity metrics that have been formulated and we found very strong correlations between these objective metrics and the subjective metrics. And actually, the subjective metrics were mediating the effect of the objective metrics on all people shows in the end, showing that they captured very much what the objective differences were and how much they affected the final choice. Once you have that established, you know, okay, this diversity metric resonates very well with my subjective diversity, so next time I can just use this metric and I don't have to ask the user again. Yeah. So I think doing this would be very useful. Yeah, you're asking.
I have been very cautiously following you for the past minutes since I have, I think, never experienced someone explaining so smoothly and so, yeah, naturally kind of the next topic that I wanted to head over and you just slightly headed over to it in a nice manner by going through the single steps without me even noticing for the first couple of minutes, but then over time thinking, oh, I guess I remember what you are now going through because this is actually the user-centric framework. Yeah, yeah. Without even mentioning that word, I was, oh, okay, that makes sense. Yes, from the system over to the perception, the experience and the interaction, ah, there we are.
And now it makes also sense because I somehow am more and more enabled to follow a kind of your traveling journey in 2016. Yeah, yeah. So a few interesting things about that paper, I think.
It was actually Michael's idea to write that paper and only afterwards I realized that, yeah, for me it felt like stating the obvious because I come from a different, I come so much from a user perspective and trying to understand human decision making, yeah, that all these things are obvious. If you ask any psychologist, they will think this is obvious. But I think the power of the papers actually that we bring it in a language that is also understandable by computer scientists. And then again, not many people in psychology actually believe that you can use interactive systems or recommender systems to achieve those goals. So there's a big gap between those fields. And I'm sort of in the middle. Very diplomatic. Yeah, but so it's interesting that for me it's so obvious that I never considered actually to write a paper until Michael was like, yeah, we should really write this up. You were like, but why this is obvious?
As soon as I realized when he made that point that it's actually not that obvious. But as always, we will include all the material in the show notes and there are slides on Slideshare and also the related paper that we will include there. And then you can also go back there, listen to that part and follow through the framework yourself. But I already think that Marte and you did a very great job in going through it without even needing the supporting graphical representation. Nevertheless, it's definitely helpful. And it's also helpful, because actually it makes me come up with follow up questions, which of course is important for the moderator. Yeah, and I was actually thinking, I'm not even sure whether I answered your original question, but let's go ahead. The original question that I, to be honest, have almost forgotten, but this actually hasn't reduced what you said in any point. So everything's fine. I guess you answered it, but still, I can't remember it, but I just feel like you answered. Okay. But actually, what I wanted to ask, I'm right now looking at it, we do have the system, we have the perception of the system. And then perception and experience are two different things. And then the experience goes into interaction and back. But there are also two things, if you look at it at the top and at the bottom. And would be great if you could touch a bit on them. So what about the situational characteristics and the personal characteristics that it seems to me like influence, perception, experience and interaction there? Yeah. So the framework indeed has this chain going from, okay, you change something about the system, the objective system, as such we call them, which leads to different perceptions, which leads to different experiences and interaction with the system. And indeed, experience and interactions are correlated. You probably behave differently if you're more satisfied. So you see actually the arrows in that model going back and forth. But indeed, those relations, so how much your perceptions influence your experience might differ depending on the context or your own personal situation. So we distinguish between personal characteristics. For example, if you are an expert, or a real movie lover, you might take recommendations differently than as an office. Actually, in a lot of our studies, we actually find that experts are typically not so happy with recommendations because they know very well what they like. It's more easy to be wrong with an expert. So that's a personal characteristic that would influence basically the relation between, in this case, probably maybe the either the perceived quality or the relation between the perceived quality and people's satisfaction. But also, for example, diversity, if you're more an expert, you might, it's easier for you to see the diversity in the recommendations. You might be a person that has a need for control.
There's actually a skill for that. And that means if I actually testing a particular interaction in my system, and I actually measure people's perceived control and how that relates to satisfaction, if I'm a person that is high in need for control, I probably the relation between perceived control and satisfaction is going to be stronger, right? Because you probably are more satisfied if you perceive that you can control the whole thing. So those are the personal characteristics. And then there's situational characteristics that the particular context in which you do this might influence those relations as well. Situational characteristics are somewhat different. They are typically are the context that might influence the relations between the subjective constructs. If you have a particular goal with the system, you might like diversity more or less. If I'm in the exploring mode on Spotify, I'm really happy if Spotify gives me very diverse Discover Weekly. But if I just want to zoom, I'm actually going to working on a paper, for example, and I need some music to concentrate, then I might want to have a very different type of diversity in my recommendation list. That's how situational characters might influence that.
Also reminds me so maybe not only with regards to the content within the single item type, but maybe also across different item types. So thinking Spotify again, I mean, I usually listen to my daily podcasts, news podcasts in the morning when I go out for a run.
And very, very solemnly to songs. So I would somehow expect then that my one or two news podcasts that I'm usually listening to would be shown at the very top and not maybe some very new diverse playlist, for example. Yeah. And of course, we have context severe recommender systems that try to do that. But this framework would actually allow you to measure that more precisely, I think. But I think we haven't actually done a lot of studies where we looked at situational characteristics, to be honest. But we have had quite some personal characteristics influencing our systems. Expertise is definitely one in different ways, like especially for example, in music. There's a lot of people. He is doing it again. I like it.
What do you mean? I'm zooming into the next topic, you mean?
Yeah, yeah, yeah. It's really perfect.
So I'm taking away all your britches.
I'm not actually mentioning that you try to intend that. Yeah, I was actually about to ask where we can maybe have actually an example of that. And I guess there was a paper that was published at RecSys 2022 by you and one of your students, I guess, Yiliang. Yeah, Yiliang, yes.
Yiliang that you presented at RecSys 2022 in Seattle. And that was actually about music genre exploration, which was also touching partially on the aspect of expertise, right?
Yeah. So please go ahead, let us know what you have been exploring there and how it fits into that user centric framework. Yeah, so the goal of this USB CTs was about how to help people discover new things or move forward, basically, we chose music as a domain, and we chose genre exploration as a topic. So how can you help people to learn a new music genre in a personalized way?
So we build a recommender system actually that recommends you music from a genre that you select that you'd like to explore. But we try to make sure that the music that we recommend from the genre in some way fits with your personal preferences. Spotify has these audio features that describe the music and we use these audio features actually to make sure that, okay, I'm a very much a classical music lover. If you want me to learn more about reggae, which is quite different, you might feed me some acoustic reggae music. Right. And that might actually trigger me because that's sort of that part of this, it's really fits with my preferences.
And this is a nice way for me to learn about this genre. Now we actually use this framework a lot in those studies because we tried a bunch of things. And for example, we also gave users a lot of control and a nice visualization and then you want to know whether this visualization is actually helpful. So we actually asked questions about the helpfulness of the visualization and found that indeed, if they think visualization is more informative, then they find the recommendations also more helpful. Those sort of relations. I think your point was about personal characteristics.
And one of the interesting things we found in this in all our studies, but we are not the only ones, actually, a lot of music recommended studies find this that expertise is musical expertise has a very strong influence on this in different ways. So first, and the nice thing is there's a very good measure of musical expertise. It's called the music sophistication index. And it measures a bunch of different things to that we use are the musical engagement score, basically.
So the active engagement score. So whether you actively engage in music related activities, for example, going to concerts, listening to a lot of music and stuff like that.
And the other one was more the emotional part. So how much does music emotionally affect you?
And especially that first one, but also the second number, especially that first one, whether you actively engage in music is very predictive on what sort of music you like.
And also very stable your preferences actually are how stable your music preferences are, and how easy it is to or hard it is to actually push you or at least help you to discover a new music genre. So one thing we did here was when people start using the system, they get a list of genres to explore, we can order them based on your current preferences. So I like classical music. Now, you can give me, for example, country music as a genre, which probably will be close to classical music. But you can also give me the reggae or the electronic music, which is very different.
And I can choose which genre to put first in the list. Now, if I want you to explore a bit further, then I push the ones that are the most different from your current preferences, right?
So we change that order to push people to explore a bit more. This is called nudging, but it's actually a personalized nudge because it's that list is different for every user of our system. Yeah, and that works really well, but not so much for the people with high expertise.
Okay, so if you are a novice, if you're not very actively engaged in music, you take those recommendations and you just pick the genres that are on top of the list, which are the most far apart from your current preferences. And you explore in a very different way. If you're an expert, you're not sure if we're going to like reggae. So let's stick with country if I'm a classical music lover. So that nudging works differently for people with higher or lower expertise. That was one thing we found actually, which is really interesting. I think there's another thing we found in analyzing a lot of the data that you collected over a bunch of user studies, where you actually could correlate the musical expertise to their users listening preferences and songs they listened before. And Spotify actually gives you short, medium, and long term preferences. So they actually make a distinction between sort of your long term preferences. So across all your listening history, what are the typical songs you really like?
So from Spotify, you get 50 top tracks from people's long term preferences, and you get a medium term, which is six months, I think, and short term, which is like six weeks or four weeks, I forgot through the public API. Yeah, to the public API. Yeah. Okay. And we actually checked, okay, how much do these long term preferences differ from medium and short term? And if you are very high on musical expertise, those differences are much smaller. So you're much more consistent in the type of music that you listen to. Yeah, which also means that it's harder to push or to push you or to help you exploring your genre. Notching. Yeah. Now, that's actually interesting, because notching is really hot in my outer field in decision making field. But most psychologists, they use notching as a one size fits all right, everyone gets the same notch. But in recommender system, we can actually personalize the notch. If we know this, we can actually make sure that that we don't push these experts too far, because it doesn't work.
I was really surprised by how these long and short term preferences differ between people with high and low expertise. And it was actually also kind of the first times or maybe not the first times, but rather rare studies that looked more at the longitudinal. Oh, yeah, of course. Yeah.
I'm right. You conducted several rounds over the course of up to six weeks, where you were interrogating the users that participated there. So can you touch a bit on that?
Yeah, so the 2022 paper at RecSys actually got the best student paper award. Because of this, actually. Yeah, basically, it was the last study of use thesis, we were actually already had done a few studies, but these were all one shot studies. So we give people a list of a list of music to listen to. And actually, we didn't even check whether they listened to it. So we asked them how much they liked it, and how helpful the tool was. But then it was over. And we were like, now, you know, if you really want to help people explore, this is not a one shot thing, right?
You listen to a news genre, and what you know, actually, whether people keep using the tool, and how useful it actually is to give them this exploration. And if we push them a bit further, is that helping? That doesn't mean that they also stay exploring longer, or do it actually fall back to their old habits or whatever. So we designed the longitudinal study. And that's indeed very rare, where we actually gave participants across six weeks, four sessions using the tool, we measured actually, over time, how this nudging of that genre early on, so you whether you get a genre that is further apart from your preferences or close, and how much it persisted, actually, and how much it helped people. And it helped a little bit. But we also saw that actually, most of the nudges fade away quite quickly. But we did find actually that people chose a genre to explore, explored it over the weeks kept exploring the playlist, they kept coming back to our system to the new session, and they kept exploring and changing the parameters a little bit, going to more personalized versus more music from that genre. And we had a slider that you could control, and they use that slider as well. And over the course of those four sessions, actually, they were really satisfied with the tool, and they thought it was really helpful.
They actually, we had very little dropout. Normally, with studies like this, you like, after two or three times, you, you, you have 20% of 30% of your participants left, but actually, we only lost, you lost like 20 or 30%. Okay, over the course of the whole study.
Yeah, over the course of the whole study, of course, we paid them what we paid them, like, it's prolific. So it was like one pound per session. So it wasn't really, any between, they had to listen to it. And they did. And the nice thing about Spotify is that we actually could use the API to then again, check their preferences, we can check, we could check back their top tracks. So right, we get from the Spotify API, you only get users top tracks.
But over six weeks, you actually influenced the short term preferences.
So the 50 tracks that you can get from people's short term preferences, you can compare the tracks at the beginning of the whole thing against after six weeks at the end.
And we found actually that people actually indeed moved a little bit towards the genre. So they actually had listened to this music so much that some of this genre bleed it into their top tracks.
Yeah, yeah. And which also means that that part of the study that you haven't had control over, but which were you were taking as kind of evidence was the Spotify recommendation algorithm, or one of them that was then coming up with that recommended songs from from the API, right, that you took as evidence. Yeah, but we, of course recommend that we gave them a recommended playlist, right? So we gave them a playlist from that new genre. And they actually listened to the playlist during the week, but also use Spotify for other means, right? Yeah, yeah. But you also actually tracked their interaction with the playlist that you recommended that you listened to.
Okay, yeah, but, but only when they use our system, we couldn't, you couldn't track their interaction while using normal Spotify. So they get the playlist into their Spotify accounts.
We asked them every session to report how much they listened to the playlist. And then of course, people indeed report that they did. But of course, you don't know how accurate that self report is.
So this is actually a case where I was really happy to actually get some real data from Spotify showing that most of our participants actually moved a little bit towards the genre that they they chose to explore, because that corroborates the notion that we found actually that a lot of things were that the nudging helped a little bit. People were actually exploring a lot with the system. But yeah, they might just be doing that because they're part of a study. But if the actual listening behavior was changing a bit as well, then we did more than just having people click buttons in the survey tool. Yeah, just maybe since I've also been tinkering a bit with the Spotify API for a project that I did two years ago, was it also at some point under consideration to somehow get access or let those users hand you over some evidence of tracks they listened to? Because I mean, under GDPR, what you can do as a user is request your streaming history track record from Spotify and maybe append this to the study. Was it something that you have considered to do? Or was this maybe a bit too sensitive or just too much effort? No, that's actually quite a good idea.
You haven't done that yet. No, during one of my classes, I actually had students also work with Spotify data a little bit as an exercise. And then some of the students actually use this indeed to get data about users preferences. But we haven't done it in our studies, but that would indeed be one way to do it. But I think it will be quite hard to convince people to go through this whole process and get all that data. Yeah, yeah, yeah, it depends a bit. There's kind of two things. So gathering your data for the past 12 months is pretty easy, I would say. Getting your whole history since you have subscribed to Spotify is a bit more of an effort. Because then again, what you do have is kind of the comparison between their self-reported listening behavior versus kind of the objective measures. So when they have listened to which song for how many milliseconds? But I guess in no point should diminish the value of that work. It's a good idea, actually, we should maybe you should do it.
No, and you touch upon a good point here, right? So as a psychologist, I do believe in user reports, because otherwise, I have nothing to go with. But I also am skeptical on how accurate this could be.
But then still, again, these are typically studies where you compare different conditions. These are basically A-B tests, right? So if you still find differences in self-reports between different versions of your system, that even if your self-report measure is not like perfect, it's still a proxy of the actual behavior. And if you see that that that proxy changes as a psychologist, I'm happy enough. Maybe as a data scientist, you're not, or as a... But that reminds me, actually, of a use case or of something that I've been working on, where the goal was not actually to be that users were successful in an objective manner. But the goal was rather that the users felt they were successful. So basically, their perception was what counted and not something objective. I mean, that could also go into a very wrong way. But I mean, if it's kind of in a balanced way, that it somewhat aligns and that users are not on purpose, false perception, this is something that I guess is a valid way to do. Yeah, I definitely agree. That is again an argument why just looking at the behavior might not be enough, right? Which brings us back to our beginning.
Behaviorism is not enough. And also to concluding this episode, just going a level higher from there, what do you see for the future regarding these works? So works that point out the importance of user centricity in terms of evaluation, looking at explicit signals at users' goals and their needs, their desires potentially. So how do you feel this is being reflected and addressed in the industry?
And in academia, so do you see that there's more traction and this gets more and more access?
What is your perception there? And what might be your wish for the future?
Or your call out? It gets a lot of traction in academia, but not so much in industry, to be honest, at least that's my perception. Right now, I was saying that Spotify is a nice example of a company does a lot of user research and it does it quite well. But still, their interface is very, it's a nice interface and they offer nice tools. But I'm always frustrated that I cannot exert a lot of control on what they're doing. Yeah, now, a lot of industry often tell me, but users actually don't want control. It's only like five or 10% of people that actually say they want this. But then my students in my class actually have taken the Spotify case as a case for a UX design project, and then they start with user needs and they talk to users and what they would like about the Spotify.
They came up with a different answer. Yeah, so when they talk to users, a lot of users say, actually, yeah, you would like some control. I think the problem is that, of course, we don't want control all the time. We use these tools. In a lot of cases, we use these tools just for simple playlists. Our goal is not with the Spotify system, our goal is somewhere else, and we just need background music or whatever, or dance music on a party or whatever.
And of course, then you don't need this. But I think the trick is that, and then goes to my wishes, actually, I think the trick is that you want to shape the interaction and the control in such a way that it fits with what users want and how they want to interact with the system.
So if you hide the control, if you give them a complex display with a lot of sliders, yeah, sure.
That's not going to work. But hey, if you have a mood playlist and you actually give people a slider to change the mood a bit, like to be more positive or negative, whatever.
Yeah. But then again, you need to know the user needs and the user goals, right?
But I get a lot of students that say, yeah, I wish I could sort of change the energy of the system or I could change the mood of this playlist a bit. I think you can design tools that do this, but then you need to go to the users, you need to test these designs with users, and develop them such that they fit with their mental models. And that's hard, because it actually requires you that you also understand a little bit better how people's brain works, how their system works. But that knowledge actually might help you in developing better tools. And if you use the right methodology to test it, you might actually find that there's very interesting simple interaction mechanisms that would enhance a lot how people experience the system and the usefulness of the system. Because it's actually a bit disappointing that we have those recommender systems, and it's easy to give a lot of control, right? It's easy to change the diversity. If your music, it's easy to change a bunch of the important characteristics like energy of the music. We don't offer these things to users. That remembers me of a couple of things where this has also been done or implemented successfully, because sometimes the way of doing this can be even more lightweight, even though I go with your slider example, but it could just be the possibility to provide negative explicit feedback, like you could do on Instagram, when you say, yes, this ad is not relevant for me, because I have already bought that bad sheets or something like that. Or if it's on LinkedIn, and you say, this is not relevant for me, and you're getting asked, is it because of the person who's posting this? Is it because of the content? So very easy ways of explicitly saying this is not relevant for me, or this is something I dislike. And actually, I mean, this podcast has been implicitly making a lot of positive advertisement for Spotify. Now also to bring up some criticism here as a user.
Since I've been recently just experiencing some bad experience on actually two sides. So actually, there were some playlists, there was just recently carnival in Cologne, and of course, I had to play some carnival playlist, and was really like, I wanted just to play that playlist. And at some point, it was somehow mixing with some weird party music. And I was like, no, I just wanted to listen to that playlist, please play that playlist. I wasn't somehow possible, maybe I was just too dumb to provide that feedback. And also what I've been recently observing is kind of the auto continuation of podcasts. So I'm listening to podcasts quite often, and to many of them in kind of the finance news economy realm. And sometimes there are podcasts, which I definitely unfollowed, which I don't want to follow anymore. And they are just slide it into my auto continuation with no, I don't want to listen to that.
No, exactly. So the negative. Yeah. And I think negative feedback is something that is implemented more and more also, the discovery has it, right? You can actually, indeed, I use the lot on Facebook and LinkedIn as well, right? This is not a post I want to see. So please, stop doing this. I also don't get the feeling it really works, to be honest, but it's because yeah, if you're locked in, or yeah, and also sometimes you actually, on the one hand, say, I don't want to see this, but you might still actively engage in that content anyway, because it's still, right? It's clickbait, it's hard to actually ignore it, right?
So then you get two signals, right? And yeah, and then also, again, it's you against a big algorithm that basically is tuned on a lot of user behavior. So, but that's more on the algorithm person to explain why this is not working. But I know that there are folks from them listening to this podcast. So yeah, maybe this might be some interesting user insights here, even though statistically insignificant, they might be still relevant.
This is what you hear a lot, right? And I think, again, this is, it's important to listen to your users, and to get in some way this feedback, but also to motivate users to actually give this feedback is still hard, right? You really need to be very much annoyed to actually start giving this negative feedback, or maybe filling in like a little drop, a little text field where you can actually say why you don't like this or whatever. So I can see that it's not often implemented, but I think the going back to the this interactive aspect, I think one thing we didn't discuss, and I'm going to try to say it very quickly, is that our preferences are quite, even for ourselves, we often don't do not know what we like. And while we already said that in the beginning, while we are listening to or looking at items or whatever, we get a better idea of what we currently like. I think you can shape the interaction also around that. So yeah, what are then, for example, having a slider, you could actually give people the choice between sort of four very different types of music that you might currently think that this user might like. And then you click on one type, and you start recommending from there, right? So you make you do part of the preference elicitation, part of measuring what people currently like, you make that part of the interaction. That's one idea that I have, and we had tried some of this, but it's still sort of on my list to do a better job in running a study showing that you could smoothly combine the interaction with better understanding your user, feeding the recommender system interactively with more of your information. Because if you do that, then it's also more motivating for people to actually give that feedback. Because it's part of the process, and they will see that it actually helps the recommender system to understand better. And you gave a nice example about the carnival music. So we also had carnival deinzhoven. I'm not a big fan of carnival, but it was hard to avoid it. Yeah, you will like a carnival playlist a lot during this week. And then of course, same with Christmas music. And of course, this contextualization, people recommend the systems take care of Spotify is not recommending you Christmas music in the summer typically, right?
But you could again make this an interactive thing, right? Spotify could actually recommend, given your current, what it does, what your preference like, okay, hey, it's carnival, do you want to hear a lot of carnival music this week? Or actually, do you not want to hear a lot of carnival music this week? Right? Yeah, yeah. That's a simple question, right?
It would be a yes, no, or like this or that question. Yeah. And it would help feed the interface in the system with a lot of knowledge, and take that and then and don't, yeah, and try to stick to it. This is the principle of reciprocity that you're touching on there. So I'm more willing to provide feedback and feed the system with self reported stuff. If I also see that this is having an effect and kind of returning to me in terms of an improved user experience. I'm not even sure whether to label this explicit or implicit feedback anymore, then because if I'm clicking on particular types of music that I might like to listen to, it's also just clicking on music, right? So it's also implicit, but it actually gives the system a lot of very clear explicit feedback about my current likings. Yeah. So that solves sort of the problem of this.
This is this problem between, okay, I want to ask the user versus yeah, I might want to infer from the behavior because that's easier and it's a more clear signal. I think that we nicely come back then to where we started the whole discussion. Full circle. Full circle, yes.
Yes.
Oh, great. Okay. Very interesting insights and thanks for sharing all of these experiences, all of the work that you are doing there. Yeah. Also with that, looking forward to many upcoming and new episodes. And at the beginning, I've already said that there are already a couple of participants and guests that I'm looking forward to welcome on the show. Are there some people that you would like me to invite to the show that you are thinking about? I guess you have also been mentioned before by some of my guests. Yeah. I'm sure it was definitely the case. I think it was long overdue to do that interview. But who do you think about? So I think Michael mentioned me.
And at the time he also mentioned Alan Stark, who used to be my PC suit and he worked on these energy recommender systems. But he's now an assistant professor at UVA in the more in the communication department. So he could also bring some nice additional perspective. So I think that definitely would be a nice person to interview as well in the future. Cool. Great. So I'm just recommending an old recommendation. Okay, great. That's totally valid. Great.
Great. Yeah, Martijn, thank you very, very much for taking part in this and for sharing all of that. It was definitely very insightful. I really appreciate it. It was also fun.
So it was a pleasure. Also your way of collaborating was great. Thanks.
Thanks a lot for the good questions. It really helped to make a nice conversation.
Thank you. Even though I couldn't convince you to become a fan of Carnival, but I also didn't do my best. So maybe I will try someone again.
Maybe at RecSys this year, I will just change properly and wear one of my costumes.
Yeah, but I'm definitely going to see you at the karaoke session. I was a bit afraid that you would actually make public that I like karaoke, but it's actually hard. So basically, I think it's all over Twitter that I like karaoke or X now. So it's hard to actually ignore the fact that there's clear evidence that I like karaoke. So I hope we can sing a song together at RecSys this year.
Let me try. I hope this time maybe it's possible to sing also a Greek Shavai for the insiders here. Or I have just to resort to something simple like Lamentary.
Yeah. Cool. So thank you again and hoping to see you at RecSys and see you soon. Okay. Bye bye.
Thank you so much for listening to this episode of RECSPERTS, Recommender Systems Experts, the podcast that brings you the experts in recommender systems.
If you enjoy this podcast, please subscribe to it on your favorite podcast player and please share it with anybody you think might benefit from it. If you have questions, a recommendation for an interesting expert you want to have in my show or any other suggestions, drop me a message on Twitter or send me an email. Thank you again for listening and sharing and make sure not to miss the next episode because people who listen to this also listen to the next episode. Goodbye.
Bye.
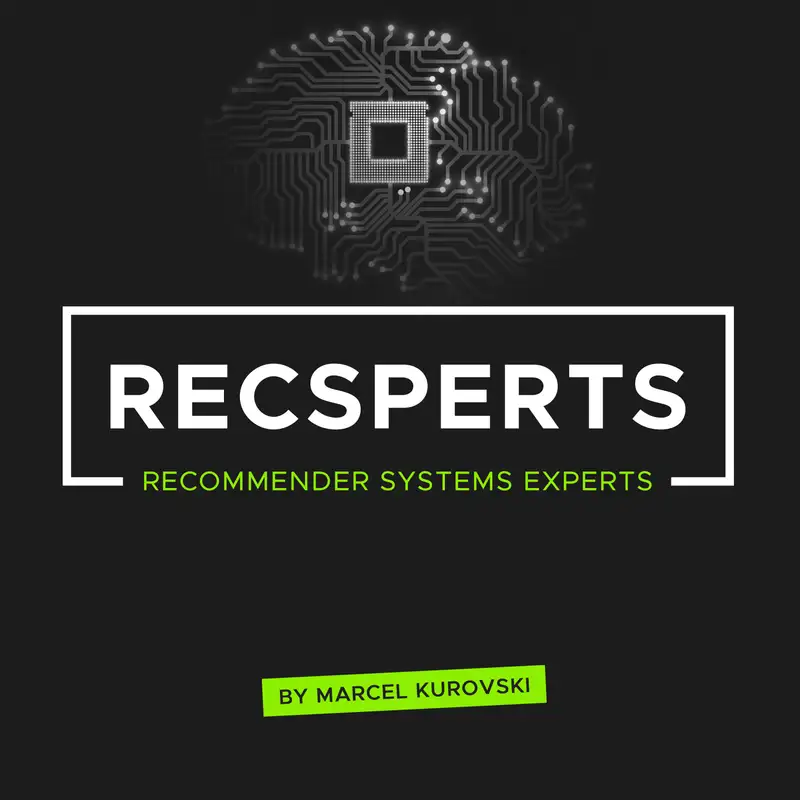